15 AI Challenges FinTech Industry Struggles With
AI is a crucial part of the FinTech industry, but it brings various challenges for businesses, making it difficult for companies to integrate this technology. We have listed the top 15 challenges in this blog.
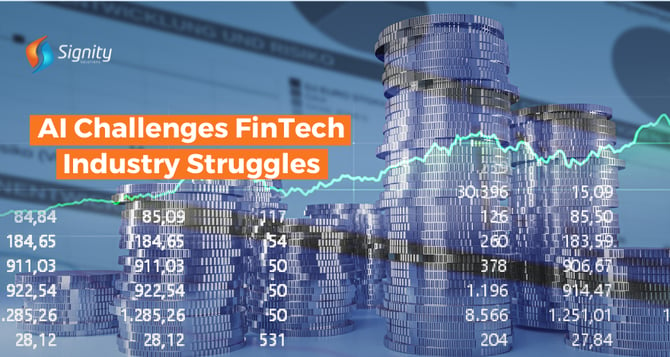
The role of Artificial Intelligence is immense in the FinTech industry. From enhancing efficiency to delivering personalized services and streamlining operations, this technology is shaping the industry.
However, AI also brings a unique set of challenges for FinTech companies, such as accurate pricing models, bias in credit scoring, and adoption of AI in the existing system. These challenges hinder operational efficiency and raise concerns about data security, customer trust, and ethical use of AI.
Working with the right AI development for FinTech services can help you eliminate these challenges for your business.
A report by Gartner states that about 90% of finance functions will use at least one AI-enabled tech solution by 2026. But along with these come the challenges.
We have listed the top 15 AI challenges in the FinTech industry; check these out.
Key Takeaways
- Artificial Intelligence is reshaping the FinTech industry by enhancing efficiency, personalizing customer services, and streamlining operations, allowing businesses to gain a competitive edge.
- From ensuring accurate pricing models to tackling bias in credit scoring and integrating AI into legacy systems, FinTech companies face a wide array of challenges.
- Partnering with specialized AI development services helps FinTech companies overcome challenges like regulatory compliance, ethical data use, and secure system integration.
- As per Gartner, nearly 90% of finance functions will integrate AI solutions by 2026, highlighting the need to address current challenges to capitalize on AI's transformative potential fully.
Top 15 AI Challenges in FinTech
Navigating the complex landscape of regulatory compliance, data security, and ethical AI deployment will be critical challenges for FinTech companies as they integrate artificial intelligence into their operations by 2025. Some other major challenges include:
1. Algorithmic Trading
AI-powered algorithmic trading automates transactions by analyzing market data and executing trades at optimal moments. By analyzing large volumes of transactional data in real-time, AI can identify patterns and deviations that humans might overlook. Its predictive capabilities also help FinTech companies proactively mitigate risks before they escalate. Key challenges include:
ML and AI models:
Making sure they can adjust to changing market conditions without performing poorly or overfitting.
Synthetic Data:
Producing accurate training data while maintaining realism.
Spot FX Transactions:
Using neural networks and preset rules to manage the intricacies of foreign exchange transactions with greater accuracy.
Decision Trees and Support Vector Machines:
Implementing these effectively for fast and accurate trading decisions.
Custom Trade Services:
Tailoring offerings to satisfy a range of customer demands while preserving scalability and speed.
2. Anomaly Detection
AI is essential for identifying irregularities that could indicate fraud or other odd activity. Instantly detecting anomalous cash payments and account takeovers through the analysis of massive transactional data makes fraud detection possible. AI reduces false positives by utilizing customized risk indicators, guaranteeing precise notifications for actions such as network hacking and money laundering.
Its integration with digital signatures enhances security, while advanced algorithms mitigate bias in AI models and protect against cyber-attacks. However, FinTech companies face challenges like:
False Positives:
Finding a balance between detecting threats and preventing pointless alerts.
AI model bias:
Minimizing data biases that could cause some fraudulent activities to go unnoticed.
Real-time Notifications:
Making sure that warnings get on time without overloading teams with instances that are not urgent.
Transaction Monitoring:
Effectively managing data while continuously keeping an eye on a large number of transactions.
Abnormal cash Payments:
Finding odd cash flow patterns without interfering with business as usual.
3. Anti-Money Laundering (AML)
AI helps strengthen anti-money laundering measures, but FinTech companies struggle with:
AI-driven Security Measures:
Develop trustworthy AI models to identify questionable activity instantly.
Risk evaluations and scores: Correctly and consistently allocating risk levels to transactions.
Threats to Cybersecurity:
Preventing sophisticated cyberattacks from compromising AI systems.
Retail and Business Clients:
Distinguishing between authentic and fraudulent actions across a range of clientele.
AML AI from Google Cloud:
Making efficient use of tools like Google Cloud while guaranteeing a smooth connection with current systems.
4. AI in Pricing
AI has revolutionized pricing strategies, enabling FinTech companies to optimize their pricing models using real-time data and dynamic algorithms. Though AI will save banks $900 million in operational costs, however, challenges arise in:
Data-driven Pricing:
Ensuring that data used for pricing decisions is accurate, complete, and updated regularly.
Market Activity Analysis:
Analyzing fluctuating market trends in real-time requires robust AI models and reliable data streams.
Personalized Pricing Strategies:
Tailoring prices to individual customers demands integrating AI with human expertise to avoid perceptions of unfair pricing.
Scenario Planning:
Predicting market shifts and adjusting strategies without introducing bias or inaccuracies.
Transaction Records:
Accurately incorporating past transaction data into AI-driven pricing recommendations while ensuring compliance with data security regulations.
5. Biometrics
AI-powered biometrics is becoming central to secure FinTech operations, but companies encounter hurdles in:
Multi-factor Authentication:
Ensuring biometric solutions complement other methods like passwords and OTPs.
Facial and Voice Recognition:
Balancing accuracy and privacy concerns, especially in diverse user demographics.
Biometric Data:
Safeguarding sensitive data from breaches and ensuring compliance with regulations.
Banking Experiences:
Delivering smooth and secure authentication processes without creating friction for customers.
Related Read: Finance and AI-Applications, Benefits, Technologies, & Implementation
6. Centralized Use of Generative AI
Generative AI holds immense potential, but centralized implementations face challenges like:
Outdated Banking Applications:
Integrating generative AI systems with legacy infrastructures.
Data Management:
Ensuring financial data is organized and accessible for AI capabilities to function effectively.
Retrieval-Augmented Generation (RAG):
Implementing RAG frameworks to enhance AI-driven customer experiences.
Synthetic Data:
Leveraging synthetic data to train models while maintaining authenticity.
7. Chatbots and Virtual Agents
AI-powered chatbots improve customer service by delivering instant responses and streamlining customer support automation. With ChatGPT integration and examples like Capital One Eno, Citi Bot SG, and TD Canada Trust Clari, these virtual agents enhance customer experience (CX) by offering personalized financial services across mobile investment platforms. But FinTech companies struggle with:
Customer Experience (CX):
Ensuring chatbots understand nuanced queries and respond effectively.
ChatGPT Integration:
Integrating advanced conversational AI like ChatGPT without significant downtime.
Virtual Agents:
Balancing automation with the need for personalized human-like interactions.
Customer support automation: Managing complex queries that chatbots may not be equipped to handle.
8. Cloud-First Architecture
Shifting to a cloud-first approach brings scalability but also challenges, such as migration from on-premises systems to platforms like AWS, Azure, or GCP. Banks and FinTech companies must adopt a hybrid approach to balance on-premises and cloud services while ensuring scalable apps address FinTech challenges without compromising performance.
Migration from On-Premises Systems:
Transitioning legacy systems to the cloud without data loss or downtime.
Hybrid Approach:
Balancing on-premises and cloud solutions for optimal scalability.
Scalable Apps:
Ensuring FinTech applications can handle growing user demands without performance lags.
9. Code Assistants
AI-driven code assistants help FinTech developers, but challenges include:
Legacy code Translation:
Accurately modernize older code bases without losing functionality.
Model Customization:
Adapting code assistants to specific FinTech development needs.
Low-code and no-code Platforms:
Striking a balance between simplifying development and maintaining robust capabilities.
10. Credit Scoring
AI improves credit scoring accuracy but faces challenges such as eliminating bias in algorithms that could affect fairness and the need to process large datasets efficiently. By incorporating machine learning trends and predictive models, AI can enhance decision support systems and better analyze spending patterns while also playing a key role in fraud detection.
Bias Elimination:
Ensuring credit scoring algorithms do not disadvantage specific demographics.
Large Datasets:
Handling and processing vast amounts of data efficiently.
Decision Support Systems:
Building systems that integrate seamlessly with financial institutions' operations.
11. Customer Sentiment Analysis
AI-driven sentiment analysis improves customer understanding, but:
Sentiment Scores:
Interpreting data accurately to gauge customer satisfaction.
Service Tailoring:
Adjusting offerings based on customer feedback while maintaining profitability.
Text Analysis and Topic Identification:
Processing unstructured data like reviews and comments effectively.
12. Increased Regulations
AI in FinTech is under scrutiny due to increased regulations, including:
AI Ethics and Transparency:
Demonstrating compliance with frameworks like the AI Bill of Rights.
GDPR Compliance:
Ensuring data security while leveraging customer insights.
Regulatory Bodies:
Staying updated with evolving industry standards globally.
13. Open Banking
AI facilitates open banking but poses challenges such as:
Security:
Safeguarding customer data while enabling third-party access.
Data sharing:
Ensuring seamless integration of APIs without compromising privacy.
Real-time Monitoring:
Continuously tracking transactions across platforms for anomalies.
14. Personalized Cross-Selling
Though it struggles with hyper-personalization, AI makes it possible to provide customized financial recommendations while guaranteeing that solutions satisfy particular client needs without infringing on privacy rights.
Although customized AI models can offer financial advice and product recommendations, there are difficulties in maximizing deployment tactics and efficiently utilizing open-source tools to expedite loan approval procedures and fraud detection.
Hyper-Personalization:
striking a balance between privacy concerns and personalization.
Strategies for Deployment:
Expanding solutions to accommodate a range of clientele.
Fraud Detection:
Distinguishing between cross-selling practices that are genuine and fraudulent.
15. Robo-Advisors for Portfolio Management
Robo-advisors are transforming investment strategies but face hurdles such as:
Sensitive Financial Data:
Providing automated advice while safeguarding user data.
Intelligent Algorithms:
Constantly refining algorithms to take into account market developments.
Managing a Personalized Portfolio:
Tailoring investment strategies without sacrificing automation.
Eliminate Your AI Challenges With Our Experts at Signity Software Solutions
Our team specializes in addressing the complexities of AI adoption in the FinTech industry, from ensuring compliance with stringent regulations to overcoming data biases and cybersecurity concerns. With tailored solutions, advanced AI capabilities, and a focus on ethical practices, we empower FinTech companies to unlock the full potential of AI while minimizing risks.
Are You Ready to Harness the Potential of AI for Your FinTech Business?
We specialize in building innovative AI solutions tailored to the unique challenges of the financial industry.
Whether it's optimizing pricing strategies, streamlining anomaly detection, or enhancing customer experiences with AI-powered tools, our experts are here to help you navigate every challenge with precision and efficiency.
Frequently Asked Questions
Have a question in mind? We are here to answer. If you don’t see your question here, drop us a line at our contact page.
What are the Biggest Challenges FinTech Companies Face when Implementing AI?
FinTech companies struggle with issues like data quality and bias, regulatory compliance, ensuring ethical AI practices, managing cybersecurity risks, and integrating AI technologies with legacy systems.
How Does AI Impact Pricing Strategies in the FinTech Industry?
AI optimizes pricing by analyzing market conditions, consumer behavior, and transaction records in real-time. Still, challenges like over-reliance on data-driven pricing and the need for human oversight persist.
What are the Key Concerns with AI in Algorithmic Trading?
Challenges include managing data accuracy, ensuring the reliability of AI models like neural networks, mitigating risks associated with predefined rules, and dealing with market anomalies that algorithms might fail to detect.
Why is Anomaly Detection Critical in FinTech, and what challenges come with it?
Anomaly detection is crucial for identifying fraud and irregular activities, but challenges like false positives, bias in AI models, and adapting to evolving cyber-attack strategies make it complex.
How do Increasing Regulations Impact AI adoption in FinTech?