AI in Manufacturing: Use Cases, Benefits, Challenges, and Future
Are you facing problems with your agricultural business? Did you know that Artificial Intelligence can streamline processes and enhance productivity? Check out the top use cases of AI agriculture, its benefits and challenges.
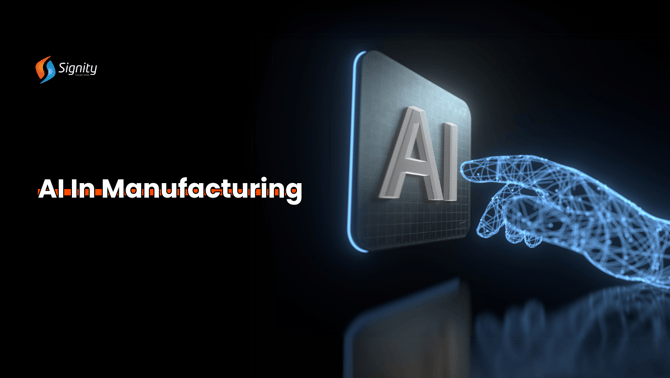
The manufacturing industry is complex, dealing with evolving customer demands, competitive pressures, and increasing operational complexities. It is difficult to manage various aspects manually.
In order to ensure a smooth process, AI in manufacturing services plays a crucial role. It provides solutions that make the operations easy and error-free.
With custom AI in agriculture solutions, businesses can deal with problems that act as a hindrance to their growth. Explore the top use cases, benefits, challenges, and future trends of AI agriculture.
Key Takeaways
- AI in agriculture: Boosts productivity with solutions like predictive maintenance and quality control.
- Key benefits: Improves inventory, forecasting, and order management efficiency.
- Challenges: Faces skill gaps, data issues, and cybersecurity concerns.
- Future trends: Innovations like autonomous systems and predictive analytics drive smarter farming.
Use Cases of AI in Agriculture
Artificial Intelligence has several uses in the agriculture sector, from predictive maintenance to computer vision, process optimization, and more. The market size of AI in agriculture is predicted to reach USD 230.95 billion by 2034.
Here are the top use cases of AI in agriculture:
Predictive Maintenance
AI-powered machine learning algorithms analyze real-time sensor data to predict equipment failures, reducing downtime and maintenance costs. This approach ensures uninterrupted production and extends the lifespan of machinery.
Manufacturers can allocate resources efficiently by scheduling maintenance only when needed.
Automated Guided Vehicles (AGVs)
AGVs, guided by AI, enhance material transportation within factories, streamlining workflows and minimizing human intervention. These vehicles use real-time data to navigate safely and avoid obstacles in dynamic environments.
They improve operational efficiency by reducing errors in material handling.
Cobots for Assembly Line
AI is used by collaborative robots, or cobots, to assist humans in repetitive manufacturing tasks, increasing productivity and safety. Cobots ensure flexibility in industrial processes by adapting to a variety of jobs.
The need for frequent reprogramming is reduced by their AI-powered capacity to learn and grow.
Computer Vision for Quality Control
Another great use case of AI in farming is the ability for quality control. Artificial intelligence (AI)-powered computer vision systems identify flaws and irregularities in goods, guaranteeing superior manufacturing quality.
These technologies lower error rates by analyzing visual data more quickly and correctly than humans. Manufacturers can easily scale quality control procedures by automating inspections.
Digital Twin for Process Optimization
Manufacturers simulate, monitor, and optimize manufacturing processes online by building AI-powered digital twins of their operations. Real-time troubleshooting and predictive modeling to evaluate changes are made possible by digital twins.
They also offer information to improve system efficiency and cut down on waste.
The image below shows that agricultural companies are eager to invest in Artificial Intelligence for several reasons.
Generative Design Software for Product Development
Product design is automated by AI-enabled generative design software, which creates and assesses millions of design iterations according to predetermined criteria. It leads to creative designs that strike a balance between affordability, sustainability, and usefulness.
When manufacturers incorporate innovative concepts straight into production, time-to-market can be accelerated.
Energy Management
By examining usage trends and spotting areas for optimization, Artificial Intelligence in agriculture optimizes energy use in manufacturing operations. Real-time monitoring makes it easier to identify energy waste and take immediate corrective action.
It helps lower operating expenses while advancing environmental objectives.
Demand Forecasting
Accurate demand forecasting is made possible by machine learning algorithms that examine previous data, improving inventory control and production scheduling.
Demand projections powered by AI assist in avoiding stockouts and overproduction.
Companies can improve customer satisfaction by matching production schedules to market trends.
Inventory Management
By anticipating stock needs, automating reorder procedures, and lowering the expenses associated with surplus inventory, AI enhances inventory management. Manufacturers are guaranteed to get the appropriate materials at the proper moment, thanks to real-time tracking.
By optimizing inventory levels, it reduces waste and enhances cash flow.
Supply Chain Optimization
By locating bottlenecks, anticipating interruptions, and enhancing logistics with data-driven insights, AI-driven solutions improve supply chain optimization. They make it possible to plan routes dynamically and track shipments in real-time.
Additionally, AI encourages improved cooperation amongst supply chain participants, guaranteeing more efficient operations.
Benefits of Artificial Intelligence in Agriculture
AI agriculture helps the companies in various sectors, from enhancing inventory management to accurate demand forecasting. Some of the major benefits of AI in farming include:
Enhanced Order Management
AI in order management automates order processing, which streamlines operations and lowers human error. AI guarantees precise inventory allocation and quicker fulfillment by evaluating client data. Data-driven insights make it possible to track orders in real-time and improve demand alignment.
Efficient Warehouse Management
AI in warehouse management simplifies material handling and maximizes storage layouts.
AI-powered robotics increases picking and packaging efficiency while lowering human mistakes and effort. AI-driven systems forecast inventory requirements, guaranteeing ideal stock levels and space use.
Accurate Demand Forecasting
Machine learning algorithms are used in AI-driven demand forecasting to examine past data and estimate market trends. Manufacturers can precisely adjust production schedules to satisfy client requests.
It improves resource allocation, decreases waste, and lessens overproduction.
Improved Quality Control
High product standards are ensured by AI-powered computer vision and quality control systems that precisely identify flaws. These technologies increase production speed without sacrificing quality since they operate more quickly than conventional checks. Defect identification in real time helps avoid expensive recalls and rework.
Connected Factories for Seamless Operations
AI is used in connected factories to combine workflows, systems, and machinery for real-time data transmission. Predictive analytics is made possible by this connectivity, which boosts productivity and decreases downtime. AI improves overall operational visibility by encouraging collaboration across production lines.
Optimized Supply Chains
AI-powered supply chain optimization improves logistics by anticipating interruptions and streamlining routes. Algorithms that use reinforcement learning determine the most efficient methods for managing resources and shipping costs. 72% of manufacturers reported lower costs after deploying AI.
Innovative Product Design and Maintenance
Development processes are sped up using generative AI, which automates the generation of creative product concepts. Through proactive repair scheduling and machine health analysis, predictive maintenance minimizes downtime. When robotics and AI are combined, firms can increase production processes' accuracy and efficiency.
Challenges of Implementing AI in Manufacturing
Change Management and Cultural Resistance
Cultural resistance to AI implementation is common because workers may find it difficult to adjust to new technologies or fear losing their jobs. Effective change management techniques are required to promote acceptance and guarantee a seamless transition.
Skills Shortages and Improper Training
The shortage of qualified personnel creates significant obstacles to administering and implementing AI systems. AI technologies may be underutilized as a result of inadequate training of current employees.
Data Quality and Availability Issues
High-quality, easily accessible data is essential for AI systems, but deployment is frequently hampered by fragmented and siloed data. Unreliable AI results can result from inadequate or inconsistent datasets.
Technology Complexity and Investment Roadblocks
Integration might be intimidating due to the technological complexity of AI systems, particularly for complicated task automation. Barriers to investment and high upfront expenses could deter companies from fully implementing AI.
Cybersecurity Concerns and Explainability
AI integration raises additional cybersecurity issues since linked systems are more susceptible to intrusions. Building confidence in automated decision-making processes requires explainable AI.
Future Trends of AI Agriculture
With advancements like AI-enabled robots and autonomous systems that improve accuracy and efficiency, artificial intelligence in manufacturing is poised to have a revolutionary future. Predictive maintenance and predictive quality assurance will be made possible by the combination of deep reinforcement learning and sophisticated neural networks, which will reduce downtime and faults.
Explainable AI (XAI) provides transparency to decision-making, whereas generative AI will transform design processes. The growth of the smart factory will be facilitated by the democratization of AI, which will make advanced technologies available to all manufacturers. Businesses will remain ahead of the competition with strong AI product strategy and developing machine learning algorithms.
Build Custom AI Agriculture Solutions With Us
Ready to enhance your business growth with tailored AI solutions for your agricultural business? Meet our experts at Signity Software Solutions to discuss your needs and get the right solutions for your business.
Artificial Intelligence is the Future of Farming
Connect with us to explore smart solutions that improve efficiency and reduce costs.
Our AI experts have years of experience in the agricultural sector and will work with you to build the best solutions that deliver the desired results quickly. Connect with our team today.
Frequently Asked Questions
Have a question in mind? We are here to answer. If you don’t see your question here, drop us a line at our contact page.
What is the Role of AI in Manufacturing?
AI in manufacturing optimizes production processes, enhances efficiency, reduces downtime, and improves quality through data analysis and automation.
What is the Future of AI in Manufacturing?
The future of AI in manufacturing involves increasing automation, predictive analytics for better decision-making, and enhanced operational efficiencies, thereby driving innovation and sustainability across the industry.
What Challenges do Manufacturers Face when Implementing AI?
Common challenges include cultural resistance, data silos, skills shortages, cybersecurity concerns, high initial investments, and the complexity of integrating AI technologies.
How Does Predictive Maintenance Benefit Manufacturers?
Predictive maintenance leverages AI to analyze machine health, detect potential failures, and schedule repairs, reducing downtime and maintenance costs.
What is the Future of AI in Manufacturing?
The future includes smart factories, generative AI for product design, deep reinforcement learning for autonomous systems, and advanced neural networks for real-time optimization.