How Custom LLMs Can Transform Your Industry?
Right from data processing to the techniques used for customization, learn all about custom LLMs in this blog. This blog will also help you learn about the challenges of implementing a custom LLM, like security risks and resources. You will also learn about the best practices for custom LLMs and how a development firm can assist you.
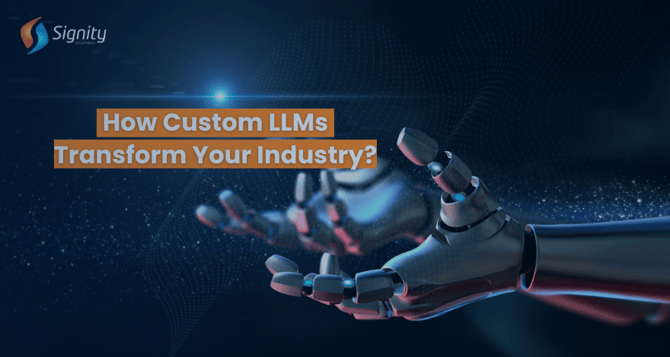
If you are a business owner, here’s a quick question for you: How can you make your LLM model more attuned to your business needs? The answer lies in customizing the LLM or deploying custom LLMs.
According to this report published by Datanami, nearly 67% of organizations have incorporated large language models into their workflows and are utilizing GenAI capabilities to unlock unprecedented insights, automate tasks, and optimize processes.
A Custom LLM helps address an organization's specific pain points. While building your very own LLM, it is important to keep in mind the subtle customizations. This will help the LLM better understand your business needs.
Key Takeaways
- Custom LLMs are being implemented by many companies today because of the vast number of use cases.
- The key features of a custom LLM include personalization, domain-specific integration, contextual adoption, and optimization.
- There are numerous cases of custom LLMs being used in industries like legal services, the healthcare sector, and education.
- There are challenges while implementing LLms, but they can be handled if the firm follows good practices, like cybersecurity precautions.
What Are the Key Features of Custom LLMs?
With the key focus on personalization, domain-specific integration, contextual adaptation, and optimization, LLM development unveils a transformative journey in AI advancement.
Let's delve deeper into the critical components driving the evolution of LLMs and see how they work together to make custom LLMs so powerful:
Personalization
Personalization is crucial to LLM development. LLMS are extremely important as they adhere to a business's specific use cases and maximize its profit. LLMs, in this case, require a lot of data, like product information, company policies, and the particular technology being used to build and start functioning according to the use cases.
Domain-specific Integration
Data sets are concentrated in their respective fields. The LLMs used for domain-specific integration are trained using these data sets. Every organization has pre-set guidelines, and these domain-specific LLMs adhere to these guidelines strictly. They differ from foundational models in their training processes. Unannotated datasets are used to train foundational models, while annotated datasets are utilized in the case of domain-specific LLMs.
Contextual Adaption
If you give a few examples to a Large Language Model, it will perform according to that context. This is called the contextual learning ability of LLMs. Analogies are the basic building blocks when it comes to contextual learning.
There are various approaches to this in the field of AI, like Chain of Thought (COT), which involves improving the capacity of LLMs to work in reasoning tasks, self-consistent COT, and Tree of Thoughts.
Optimization
At times, a custom LLM will generate incorrect outputs due to wrong prompts, text messages showing errors, or low computational efficiency. Optimization is the process of removing all the loopholes from an LLM model. As we know by now, LLMs are made up of neural networks, and in optimization, the neural network structures are tweaked. Also, during the optimization of LLms, the training processes of LLMs are refined.
What Is the Process of LLM Customization About?
Advanced AI capabilities and very specialized processes come together in the process of LLM customization. Pre-trained models are used to create LLMs, and these models are trained and tailored to meet the specific needs of the business.
Collecting and processing the data is the first step towards LLM customization. Relevant datasets are created and customized to suit the business needs. This forms the bedrock for the entire LLM customization process and sets the stage for robust learning and continuous improvement.
Once you contact a trusted LLM development firm like Signity, things become simpler. Our custom LLM solutions help improve search relevancy. We deliver personalized and insightful results after taking into account various factors, such as purchase and browsing patterns.
It is very important to get a clear understanding of the techniques used to create custom LLMs. Only then can you start developing custom LLMs.
What are the Techniques for Creating Custom LLMs?
Creating custom LLMs requires the use of advanced technologies like fine-tuning, RAG, and prompt engineering. Let us see how these work:
1) Fine-tuning
Finetuning is basically the process in LLM development through which users adapt pre-trained LLMs to tasks that are more relevant to the organization. The best practice to go about this is first to select a specific domain in which we would like to make the custom LLM work, then train the same on a limited data set.
Doing this surely gives more relevant and domain-specific results, reduces the time for application development, and brings down the costs significantly. The parameters of the pre-trained model were readjusted, and the model eventually developed a very deep knowledge of the domain.
2) P-tuning
In order to understand what p-tuning or prompt-tuning is, it is important to understand first how prefix tuning works. In full-tuning LLM development methods, a lot of parameters are introduced to the LLM model at once.
This is different in prefix training, wherein a limited number of parameters are employed. P-tuning is a simplified way of prefix-tuning itself, where the prompts are used to change specific zones of the model, unlike in fine-tuning, wherein almost all the aspects of the model are altered.
3) RAG
Rag stands for retrieval augmented generation, and it allows users to get the maximum benefits of custom data for large language model development.
By using RAG, the user should expect more prompt responses, and the outputs often contain the source from which the information was taken, which ensures authenticity. Also, if you glance through the other procedures used to customize LLMs, RAG is comparatively affordable.
Prompt Engineering
This is a feature of large language model development and highlights AI's progress in advanced machine learning and problem-solving. With prompt engineering in the picture, AI developers efficiently generate tailored prompts to extract specific information and iterate on solutions swiftly.
This feature of a custom LLM not only expedites the engineering process but also fosters experimentation and discovery, paving the way for groundbreaking advancements across diverse domains. With prompt engineering at the forefront, large language models empower engineers to navigate complex challenges with precision and ingenuity, driving progress and shaping the future of technology.
Some use cases of Customized LLMs
Now that we have an idea about the transformative and agile nature of LLMs, it is important to look at a few use cases of custom LLMs. From customer service support to aiding in defense operations, there’s nothing that llms cannot do today.
- Customer Support
- Content Generation
- Healthcare
- Legal Sector
- Banking and Finance Sector
- Defence Sector
- Media Houses
1.) Customer Support
This is one of the most advanced use cases of custom LLMs. The main goal is to give accurate and instantaneous responses to client queries consistently. The main point is to use NLP along with LLM. Using advanced technology like NLP and guardrails helps decipher human prompts more clearly. Using personalized chatbots, firms actually respond to client queries promptly, leading to increased customer satisfaction and loyalty.
Similarly, a software company deploys a custom LLM to address technical support queries, troubleshoot issues, and provide step-by-step guidance to users. By leveraging custom LLMs, businesses streamline customer support processes, reduce response times, and improve the overall customer experience, leading to higher satisfaction levels and increased loyalty.
2.) Content Creation
Today, LLMs are being used to generate all sorts of content, such as articles, blogs, newsletters, social media posts, brochure content, etc.
One of the most sought-after use cases for LLM development is their ability to automate content generation by streamlining the generation of articles, blog posts, brochure content, ad copies, and website copies. LLMs have the unique capability of creating personalized and relatable content without fostering anomalies like keyword stuffing.
3.) Healthcare
Recently, Electronic Health Records (EHR) providers have started using LLMs to identify trends and patterns since LLMs sift through very complex data structures.
Again, LLMs skim through very elaborate patient records and are used in medical imaging for predictions. LLms are used in drug discovery since they can predict drug interactions, drug patterns, the potential risks associated with the drug, and how efficient it could be for the patient.
When it comes to diagnostics, LLMs have helped achieve 83.3% accuracy.
4.) Legal Services
In legal research, scholarly articles, law documents, and criminal justice files are all humongous documents that would require countless hours or even days for a human being to scan across. An effective LLM will do that in seconds.
They generate responses relevant to the legal domain. Litigation mining is equivalent to an advanced level of data mining. LLMs identify the evidence from case records, and advocates even use LLMs to prepare questionnaires as witnesses. They can even predict the outcomes of legal cases and aid in getting the cases solved rapidly.
5.) Banking and Finance Sector
The growing popularity of custom LLMs in the fintech domain is best understood by the response whenever you type “Fintech and LLMs” in Chatgpt or even the Google search bar.
We all know how prone the banking industry is to cybersecurity and malware attacks. Custom LLMs easily predict the risks associated with every financial move and even suggest ways to mitigate them.
LLMs are now being used to track a person’s spending habits and guide people in investing, trading, etc. They also improve the accuracy of financial decisions made by firms or institutions. The only caveat is the huge resources spent during the training phase of the custom AI models, but research on how to curb that is underway.
6.) Defense and Services
In the military, a large chunk of the training is conducted by emulating real-life scenarios. Situations are posed, and then the trainees have to find ways to wriggle out of the situation. A pre-trained LLM suggests this kind of scenario to the military planning team. LLMs are equipped with phenomenal language interpretation and translation abilities. When it comes to imparting training to people across borders or from different cultures, LLMs are a boon.
Large language models (LLMs) play a crucial role in defense by enhancing intelligence gathering, analysis, and decision-making processes. These models sift through vast amounts of text data, including open-source intelligence, intercepted communications, and social media posts, to identify patterns, trends, and potential threats.
LLMs enable defense agencies to extract valuable insights, detect emerging threats, and assess geopolitical risks more efficiently than traditional methods.
Moreover, LLMs support strategic planning, scenario analysis, and predictive modeling by analyzing complex geopolitical dynamics and simulating potential outcomes. By leveraging LLMs for defense applications, agencies strengthen national security, mitigate risks, and stay ahead of adversaries in an increasingly complex and dynamic threat landscape.
7.) Media Houses
Media houses are busy places, always buzzing with energy and chaos. There has to be minimal response time to clients, and ChatgPT helps with that. Other than that, LLMs aid in data classification and customer segmentation. They make the data more readable and assist with customer segmentation, too. When it comes to verifying or double-checking data, LLMs are a blessing in disguise. They also come up with customer retention ideas.
8.) Human Resources
LLms are actively being used in human resource departments because of their ability to identify potential candidates. Other than this, a generative AI model looks at the firm’s operations and drafts a job description for a vacancy.
When it comes to training employees, LLM development easily suggests how to go about it by studying the competitors in the market. We all know that a vast amount of financial resources is spent in selecting the right candidate to hire. A well-trained LLM model easily helps curb these expenses. Apart from this, LLMs even help assess the performance of a candidate.
What Are The Challenges of LLM Customization?
1.) Custom LLMs are biased
This is very important to take care of. LLMs often generate biased outputs, which pose many risks to users. They are usually seen to exhibit many biases, such as ethnic or cultural. For example, if ChatGPT has data showing that most teachers are women, it will start generating outputs that further fortify the assumption.
2.) Custom LLM Hallucinations
Sometimes, due to the complexity of the model or biases present in the model, an LLM generates output that, despite being grammatically correct, may need more sense and meaning. This is called an LLM hallucination. In fields like health, legal service, or science-related fields, this alone will lead to a catastrophe.
3.) Resource limitations
Not all LLMs are cost-effective. Some of the models, like Llama 2, are cost-effective. Some of the factors on which the price of a custom LLM depends are the size and version of the model, the frequency of computational requests made, and how much budget/ resources are allotted to each response.
Here are some ways in which one can limit the expenses in case of LLM deployment:
-
Relevant Prompts
Going for more relevant prompts, the less useless tokens a prompt contains ( like characters/ texts). The less you will be charged for your LLM
-
Caching Data
If you find a way to store the popular data or prompts that are trending on that day, it will save a lot of the cost
-
Batching Requests
Batching requests simply means clubbing requests together instead of sending them individually. Firstly, this method helps you save time, and secondly, it saves overhead costs, too.
4.) Data Quality
LLMs gather data from various sources, which often leads to inconsistencies. Secondly, the quality and the data - as in the significance and making sure it is reaching the user on time, are to be used. It is a big deal to manage humongous chunks of data while ensuring its consistency and accuracy.
Best Practices For Developing Custom LLMs
Every organization needs to know and understand the best practices for custom LLMs, which would improve its overall efficiency.
Let us have a look at some of them:
1.) Cybersecurity Best Practices
When working with LLMs, it is important always to consider the system's cybersecurity risks. Miscreants can easily break in and try to take advantage of biases. It is important to contact an AI consulting company that can help with this.
2.) Ethical Considerations
Sometimes, there are a lot of biases present in the training data of custom LLMs. This might lead to erratic outcomes and spreading of discrimination or even hatred. LLM development services should focus on the explainability and the interpreting power of llms and make sure these do not pop up during output generation.
3.) Diverse Data Sets
If only one data set is used to train a large language model, the chances of it being error-filled or biased are higher. Ensuring multiple data sources during LLM development ensures the information is assimilated from many diverse sources.
4.) Evaluation Post-Fine-Tuning
Every task or output generation has KPIs and target goals. While fine-tuning a model, performance should be gauged immediately and over some time to avoid any inconsistencies.
Now that we have read about the best practices for customizing LLMs let's look at some of the future trends.
Future Trends Of Custom LLMs
LLMs are constantly changing, and in the next decade, there will be many more trends to anticipate. Let us weigh in on the same:
Personalized LLMs
What are the key characteristics of any marketing campaign or any product? Phenomenal user engagement and customer loyalty. In the future, custom LLMs will take note of everything, starting from social media activity, browser history, behavioral patterns, customer needs, etc, and come up with more structured strategies and roadmaps.
Hybrid LLMs
These are the LLMs that generate text and other formats like images, videos, graphics, etc. Even without sufficient training imparted to these datasets, they can handle very complicated customer queries and act as a bridge between rule-based systems and data-driven approaches.
LLMs Free from Ethical Bias
These ethical LLMs will prioritize the mitigation of biases related to race, gender, ethnicity, religion, and other protected characteristics by implementing robust fairness-aware learning techniques and bias detection mechanisms during model training.
How to Get Started?
So, custom large language models (LLMs) represent a groundbreaking leap forward in artificial intelligence and natural language processing. To leverage their full potential, a firm needs to know the exact use cases in which they will be used.
Contacting an established LLM development company with at least a decade of experience, like Signity, can provide valuable insights and help them navigate the challenges. Our technical expertise consists of advanced techniques like NLP, machine learning, few-shot learning, and sentiment analysis.