How RAG Improves Large Language Models to Deliver Real Business Value
Discover how Retrieval Augmented Generation (RAG) enhances traditional LLMs by adding relevant data, context, accuracy, and real-time data access. Read our blog to learn how RAG empowers LLMs to meet modern enterprise challenges.
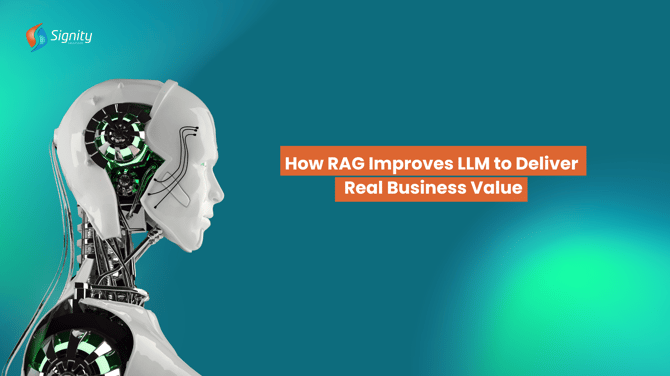
AI is changing how businesses operate. As AI capabilities expand rapidly, business leaders are adopting advanced AI models, such as traditional Large Language Models (LLMs) and Retrieval-Augmented Generation. Both utilize generative AI, but they differ in substantial ways that impact reliability, cost, and outcomes.
RAG enables traditional LLMs to tackle business challenges by basing their solutions in real-time and with better precision.
According to recent studies, the global AI market is expected to grow to over $190 billion by 2025. Traditional LLMs, such as GPT, often fall short in several crucial areas, a gap that Retrieval Augmented Generation solutions bridge by combining their fluency with retrieved, accurate information.
This blog cuts through the technical jargon to provide business leaders with a clear understanding of RAG and traditional LLMs.
Here, we’ll explore how RAG enhances traditional LLMs by providing additional context, aligning them with your operational goals, data strategies, and long-term AI roadmap. Let us begin:


- RAG enhances traditional language models by accessing real-time data. This helps provide accurate and up-to-date responses that meet the needs of businesses, making them more reliable for changing situations by utilizing the latest information.
- Businesses can benefit from RAG because it offers higher accuracy, better data security, and lowers the risk of false information. This is especially important in regulated industries like healthcare and finance.
- RAG provides the agility and reliability to align with AI initiatives that meet specific business requirements by combining real-time data retrieval with traditional LLM capabilities.
A Quick Overview of LLMs
Large language models, or LLMs, are a type of advanced artificial intelligence with remarkable capabilities. Traditional LLMs are trained on vast amounts of text data, enabling them to generate responses that are human-like. They can understand and create text that sounds like human language by examining a large amount of written material to identify patterns. People often utilize LLMs for tasks such as chatbots, language translation, and text summarization. Their success frequently relies on the quality of the training data.
Understanding Retrieval Augmented Generation
Retrieval augmented generation (RAG) is a technique that combines the strengths of large language models with the ability to retrieve relevant information from external data sources. This approach enables RAG models to provide more accurate and contextually relevant responses, particularly in scenarios where the training data may be insufficient. RAG can dynamically integrate current information, making it particularly effective for addressing real-time inquiries and engaging with users on contemporary topics.
Retrieval-Augmented Generation (RAG) enhances the way large language models (LLMs) generate responses. This process involves checking a reliable knowledge base outside of its training data for specific data before generating answers.
The RAG model excels at fetching extensive data from vast vector databases. This allows for detailed responses to complex user queries. This approach is ideal for industries such as financial analysis and medical diagnosis, where the integration of the RAG model with fine-tuned large language models (LLMs) addresses the limitations of traditional models in understanding and processing specialized terminology.
How RAG Empowers Traditional LLMs to Deliver Real Business Value?
RAG strengthens the capabilities of traditional LLMs in distinct ways, particularly by integrating external data into AI generation processes through advanced machine learning techniques. These improvements enhance the abilities of LLMs across key performance areas and business-impact parameters.
Here’s a quick glance at core capabilities, limitations, and enterprise use-case fit of both.
Parameter |
Traditional LLMs |
Retrieval-Augmented Generation |
---|---|---|
Data Handling |
Uses static, pre-trained data |
Pulls real-time data from external sources |
Scalability & Adaptability |
Limited adaptability post-training |
Highly adaptable with dynamic data updates |
Performance Metrics |
Hard to track context relevance |
Transparent, with traceable source-based responses |
Core Functionality |
Generates from memory |
Combines generation with real-time retrieval, enhancing the model's ability to handle domain-specific details |
Data Security |
Risk of outdated or unverified data |
Allows secure, curated data access |
Accuracy |
Prone to hallucinations |
Reduces hallucinations by grounded, verifiable responses |
Task Specialization |
General-purpose language output |
Tailored for domain-specific applications, fine-tuned for particular tasks |
Data Handling Efficiency in Traditional LLMs and RAG for Enterprise AI Applications
Traditional LLMs rely on pre-trained data. They generate responses based on the content windows and internal parameters, which are restricted by the token restrictions and cutoff date of their training data. This restriction makes LLMs less suitable for applications that require updated information.
RAG systems enhance LLMs by allowing them to access up-to-date information in real-time. These systems can pull information from an external knowledge base while generating responses.
The effectiveness of RAG systems relies heavily on the quality and diversity of the information sources they retrieve. This means RAG systems can provide specific and updated information without needing to retrain the model.
Having real-time access to information and generating accurate responses can be particularly advantageous in industries such as finance and healthcare, where data changes rapidly.
RAG has the ability to pull targeted and relevant information. This can enhance overall output accuracy by up to 13% compared to models relying on internal parameters.
Evaluating RAG and Traditional LLMs for Scalability and Adaptability
Traditional LLMs need a fine-tuning process and complete retraining to add new information. This process is both costly and time-consuming. It can slow response times, require substantial computational resources, and increase costs, which makes it hard to scale effectively.
Retrieval augmented generation (RAG) enables businesses to adapt and update their systems easily. Therefore, instead of retraining the entire model, RAG enhances LLMs by providing access to external knowledge sources. This makes it quick and easy to incorporate new data and also helps AI systems stay up to date with minimal effort.
Using RAG can cut costs by 20% per token. This makes it around 20 times cheaper than constant fine-tuning.
A Performance Metrics Evaluation of RAG and Traditional LLMs
When it comes to RAG models to LLMs, several factors can be considered. These parameters provide a quick clarity on how RAG enhances traditional LLMs in various ways, including the model's ability to improve performance through fine-tuning and the integration of external data sources.
A recent study published on ResearchGate provided crucial insights on both technologies. It has clearly stated how RAG improves LLMs based on relevance, creativity, and more parameters, as shown in the graph below.
This graph highlights the capability assessment of RAG models' advancement over LLMs on varied parameters. As RAG technology improves, we can expect even better relevance and creativity in the content generated by AI.
Core Functionality of LLMs and RAG Systems
The primary function of traditional LLMs is pattern recognition within their training data, which has a fixed cutoff date. Traditional LLMs are limited to the knowledge acquired during pre-training, with no ability to access new or proprietary information.
The primary function of traditional LLMs is pattern recognition within their training data, which has a fixed cutoff date. Traditional LLMs are limited to the knowledge acquired during pre-training, with no ability to access new or proprietary information.
According to Stanford’s 2025 AI Index Report, even advanced models like GPT-4 show a 25% accuracy drop when handling queries about events after their training cutoff.
RAG systems combine the generative capabilities of LLMs with dynamic information retrieval from external knowledge bases. They provide quick access to up-to-date information and company-specific content in real-time. Research from Gartner shows that organizations implementing RAG architectures see a 40% improvement in response relevance compared to standalone LLMs.
Data Security in RAG and Traditional LLMs
Traditional LLMs may be vulnerable to serious data security issues that extend beyond standard cybersecurity concerns. Most commercial LLMs rely on cloud-based APIs, meaning companies must send their queries to an external server.
These queries often contain sensitive information. This setup presents various risks for businesses, particularly in fields such as healthcare, finance, and legal services. Sending data outside the organization may violate rules like HIPAA, GDPR, or confidentiality agreements.
Retrieval-augmented generation (RAG) systems change this data security situation by altering how and where data is handled. The main advantage of RAG systems is that they can run within a company’s existing security framework. This enables the processing of queries, document retrieval, and even the language model to occur in environments that the business controls.
When choosing between RAG and fine-tuned model development, it is essential to consider various aspects, including cost implications and performance metrics.
RAG Models Boosting Response Accuracy in Traditional LLMs
Traditional LLMs possess strong capabilities, but they face a key accuracy issue that impacts business outcomes. These models have concerning rates of AI hallucination, which is a significant concern for businesses.
The problem worsens when it comes to specialized fields that need expert knowledge. A study published in Nature Machine Intelligence found that traditional large language models (LLMs) made mistakes 27% of the time when dealing with specialized questions.
RAG models enhance LLMs by providing improved reliability and contextually relevant responses, as illustrated in the graph above. RAG systems look for relevant documents or data from trusted sources before giving answers.
This AI technology significantly lowers hallucination rates. Research indicates that RAG systems reduce false information by 60-80% compared to standard large language models (LLMs). This happens because RAG responses are based on specific, verifiable information rather than just learned patterns.
By enhancing the accuracy and relevance of responses, RAG models ensure more precise outputs, which is crucial for tasks like financial reporting and semantic meaning in specialized domains.
Prioritize Accuracy in your AI-Powered Applications
Connect with the AI development experts to learn how RAG can significantly reduce hallucinations and improve the reliability of LLM responses.
Task Specialization in Retrieval Augment Generation and Large Language Models
RAG systems are ideal for tasks that require recent or specific information, such as answering questions about new research, legal documents, or medical guidelines. Their ability to access live data gives them an advantage in these situations.
Traditional LLMs are best suited for general-purpose NLP tasks, such as summarizing, paraphrasing, translating, writing stories, or providing chat-based help. It is ideal for LLM applications where real-time data is less critical.
The Role of External Data in Enhancing LLM Capabilities
External data plays a crucial role in enhancing the capabilities of Large Language Models (LLMs) through techniques such as Retrieval Augmented Generation (RAG).
By allowing LLMs to retrieve relevant information from external data sources, RAG enables these models to provide more accurate and contextually relevant responses. The integration of external data significantly enhances the performance of LLMs on specific tasks, including question answering and text generation.
The ability to fine-tune LLMs with external data allows them to adapt to specific domains or tasks, enhancing their understanding of specialized terminology and concepts. For instance, in the healthcare industry, fine-tuning an LLM with medical literature can improve its ability to provide accurate diagnoses and treatment recommendations.
How RAG Overcomes LLM Limitations for Enhanced Business Applications?
When concluding RAG vs LLMs, the real question that arises is how RAG complements LLMs with its capabilities. Not the comparison. As RAG systems augment the capabilities of LLMs, they can advance to higher levels.
While traditional LLMs offer impressive capabilities, their implementation in business contexts reveals several significant limitations that can impact operational effectiveness and ROI.
Due to its capability to generate texts that are well-written and contextually relevant, users may be misled into factually incorrect, outdated, or even entirely fabricated data. Privacy and security concerns regarding potential data leaks through large language models (LLMs) are also of great concern for statistical organizations.
Limitations of Traditional LLMs for Business Applications
- Knowledge Cutoff and Outdated Information: Traditional LLMs only retain knowledge up to the date they were trained. This creates a problem for businesses that need updated information. For example, a model trained on data up to 2023 will not be aware of any market changes, new laws, or emerging technologies from 2024 onwards.
- Hallucination Issues: One major concern with traditional LLMs is their tendency to create “hallucinations.” These are statements that sound believable but are actually incorrect, and they are often presented with a confident tone.
- Scalability Challenges: Deploying traditional LLMs at enterprise scale involves substantial computational infrastructure. This poses an issue with scaling resources as needed.
- Integration Complexities with Existing Business Systems: Traditional LLMs weren’t designed with enterprise integration in mind. This creates problems when attempting to integrate them with the existing business infrastructure.
- Specific Context Adaptation: Traditional large language models (LLMs) struggle to adapt to specific contexts. LLM fine-tuning involves adjusting the parameters of a pre-trained model on a specific dataset to tailor the model to particular needs, such as legal document analysis or sentiment detection. This enables the adaptation of a general-purpose model to excel in specialized tasks.
This is where RAG implementation can empower traditional LLMs to overcome these limitations.
RAG fundamentally reimagines how artificial intelligence systems access and utilize information, directly addressing many of the limitations of traditional large language models (LLMs) in business environments.
How RAG Addresses Limitations of Traditional LLMs?
Parameters | Traditional LLMs | RAG |
Accuracy | Low | High |
Adaptability | Low | High |
Applications | Limited | Wider |
RAG models offer significant advantages over traditional LLMs, particularly in terms of accuracy and adaptability. One key aspect is the resource intensity required to serve user queries.
While RAG models demand more computational power and memory for processing these queries, they excel in handling numerous user queries efficiently after the initial fine-tuning phase. This makes them highly effective for applications requiring high precision and adaptability.
1. Access to Updated and Company-Specific Knowledge Bases
RAG systems excel at bridging the gap between general AI capabilities and your organization's specific knowledge assets. By dynamically retrieving information from up-to-date sources, RAG ensures responses reflect the current state of product specifications and pricing, company policies and procedures, customer data, and more.
This capability delivers significant business value by ensuring access to a wider range of precise and domain-specific information. With organizations implementing RAG reports, 83% higher precision rates for company-specific inquiries.
2. Minimizing Hallucinations Through Accurate Responses
RAG systems help reduce errors by using information from retrieved documents instead of just relying on the model's internal setup. This approach offers several benefits:
- Responses can be traced to specific source documents.
- The system provides citations for verification.
- It can offer confidence scores based on the information quality.
- The model can acknowledge when certain information is unavailable instead of fabricating answers.
3. Enhancing Answer Accuracy for Specific Domain Questions
Domain expertise represents a substantial competitive advantage in specialized industries. RAG systems excel at leveraging this expertise by connecting language models to proprietary knowledge.
For instance, it can link AI systems to the latest research, treatment protocols, and patient care guidelines in the case of Medical organizations. For technology companies, they can utilize technical documentation, code repositories, and troubleshooting guides.
This domain-specific information access enables RAG systems to outperform even the most advanced traditional LLMs on specialized queries.
4. Enhancing Transparency and Explainability of AI Decisions
As AI governance requirements evolve, it is crucial to explain how AI systems generate their outputs. RAG architectures offer clear benefits for explainable AI, including improved source attribution. They allow us to trace the flow of information from source material to the final response. This transparency meets an important business need.
Minimize inaccuracies in your AI outputs
Discover how grounding large language models (LLMs) in retrieved data through RAG can substantially reduce the risk of generating false information.
Success Story: AI-Powered Chatbot for Lung Disease Diagnostics
A radiology company in Australia sought to enhance patient engagement and streamline internal processes. They aimed to create a secure, AI-powered medical radiology chatbot focused on lung diseases like COPD, pneumonia, and lung cancer.
The company required a secure solution to efficiently address patient inquiries, automate routine tasks such as appointment scheduling, provide doctors with rapid access to diagnostic tools and treatment guidelines, and ensure compliance with HIPAA regulations.
Our team developed a HIPAA-compliant, AI-powered chatbot leveraging RAG systems to offer.
- Real-time access to medical information and seamless appointment booking for patients.
- Quick retrieval of imaging protocols and patient data to support clinical decisions for doctors and medical staff.
- Automated query handling and reduced the manual load by 30% for the administrative staff.
The AI chatbot provided round-the-clock support to patients, ensured data handling met HIPAA standards, and offered a flexible system ready for broader use. For those interested in exploring AI further, learn how to use ChatGPT-4 for free.
Bottom Line
Traditional language models (LLMs) are great at generating text, but RAG takes this a step further by using specific data to enhance its intelligence.
For companies that want to move beyond basic AI and create solutions that truly use their unique knowledge, RAG can be a powerful tool. RAG has a clear advantage for businesses that need accuracy, relevance, and actionable insights.
At Signity Solutions, we understand that selecting the right AI development company can be a crucial decision for businesses. We help companies to bridge the gap between AI potential and real-world performance.
Whether you want to explore generative AI applications, build custom RAG solutions, or expand your AI system with precision and compliance, we have the expertise and resources to guide you.
Frequently Asked Questions
Have a question in mind? We are here to answer. If you don’t see your question here, drop us a line at our contact page.
What is the difference between RAG and LLM?
RAG provides the strengths of generative models and information retrieval systems. It improves the model's output by integrating real-time data retrieval to enhance relevance and appropriateness.
On the contrary, LLMs are the pre-trained models that generate text based on vast datasets without integrating real-time retrieval. This makes it more suitable for general-purpose language tasks, such as summarization or content creation.
Does RAG improve LLM performance?
Yes, RAG improves LLM's performance by enhancing its relevance and accuracy. It also improves the performance by reducing AI hallucinations. The system retrieves and injects vital context to guide the model to a correct response.
It achieves this by identifying relevant documents or information related to a question and incorporating them as context for the large language model (LLM).
When should I use RAG instead of LLM?
Can Traditional LLMs integrate External Knowledge, such as RAG Systems?
No. Although LLMs are capable of understanding and generating human-like text, they don't integrate real-time external knowledge. They only process data gathered from internal knowledge only.
Can RAG and LLM work together?
What is a RAG in LLM?