Role of LLMs in Finance and Banking Industry
Learn how LLMs in the banking and finance sector are aiding in areas like risk management, enhanced personalization, and product recommendation. Discover some major challenges faced while implementing LLMS, such as compliance standards, workplace adoption, and privacy concerns- they are a boon for the industry.
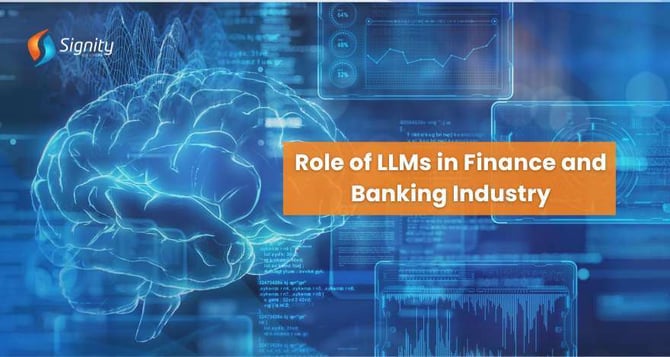
We have all heard about how big banks are leveraging large language models in their operations. Large Language Models are a savior in everything—be it analyzing huge chunks of unstructured data, risk management, or aiding in regulatory compliance.
According to a report published by the Alan Turing Institute, the rate of adoption of LLMs in finance between 2023 and 2029 has an annual CAGR of 21.4%.
In this blog, we will explore how LLM solutions are reshaping the industry.
Let’s get started.
Applications Of LLMs In Finance And Banking
From elevating customer experiences to streamlining operations and improving regulatory compliance, Large Language Models (LLMs) in finance and banking sectors are redefining how institutions interact with customers and manage their businesses.
Let's explore the transformative applications of LLMs in these dynamic industries:
Fraud Detection and Prevention -
Large language models have advanced natural language processing (NLP) abilities that help them analyze vast amounts of data. They can identify patterns and anomalies, searching for any potential fraudulent activities in text-based communication.
Traditional methods often struggle to keep up with the ever-increasing volume of data, such as chat records and text messages. LLMs, on the other hand, continuously learn and improve from new data, enabling banks to stay ahead of evolving scams and respond swiftly.
Risk Assessment and Management -
LLMs in banking help institutions consider different scenarios and assess the associated risks. They analyze historical data and market trends to flag potential issues. One of the most valuable use cases involves creating a detailed customer profile based on a customer's transaction history and online behavior. This allows for a more nuanced understanding of potential risks.
LLMs also ensure that banks adhere to the latest regulatory guidelines, such as IFRS (International Financial Reporting Standards), CCPA (California Consumer Privacy Act), and GDPR (General Data Protection Regulation).
Personalized Customer Service -
Owing to their advanced NLP abilities, LLMs in finance and banking can seamlessly process and interpret customer queries. They can even predict customer behavior, needs, and preferences. This allows banks to anticipate fluctuating customer needs, especially before significant market shifts.
Large language models can resolve many issues by understanding problems in real time. However, some aspects may require human expertise. In such cases, LLMs can seamlessly transfer queries to human agents. They also ensure productive service delivery by facilitating communication across different channels like social media platforms and websites.
Ready to Unlock the Efficiency and Customer Satisfaction Benefits of LLMs?
Don't wait to explore the potential of LLMs in your financial institution. Signity's free assessment helps you identify opportunities and develop a customized LLM strategy.
News Analysis and Sentiment Detection -
This is another important LLM use case. Large language models can sift through massive amounts of unstructured data from various sources, such as news articles, social media archives, and books. With their NLP capabilities, LLMs can perform sentiment analysis, gauging the overall emotional tone of a piece of text. They can then analyze and predict the potential impact of news articles on the financial markets and public perception.
Understanding the sentiment of a customer or a financial institution is also valuable. LLMs can help identify relevant news articles, persuasive write-ups, and case studies that could influence investment patterns. Overall, LLMs in finance and banking empower individuals to make more informed decisions.
Portfolio Management -
Large language models play a crucial role in portfolio management for banks and financial institutions. Traditionally, portfolio management relied on Large Loan Management Systems (LLMS), which focused on optimizing loan portfolios through data-driven strategies. LLMs take this a step further by utilizing advanced analytics to enhance risk assessment and maximize profitability.
In banking, LLMs can assist with stress testing. By considering various economic situations and scenarios, LLMs can predict how individual portfolios would be impacted. They can also assist banks in complying with regulatory requirements and generating regulatory reports.
Customer Support and Chatbots -
LLMs in finance and banking can analyze a customer's spending habits and financial history. This allows them to provide tailored recommendations regarding budgeting and investment opportunities. If a customer is struggling to meet their financial goals, LLMs can suggest effective strategies and solutions.
Furthermore, LLMs can recommend specific financial products that could be beneficial to the customer. They can also provide real-time alerts about pending and upcoming payments. When it comes to customer queries, LLMs can power personalized chatbots that provide instant resolution to problems.
For a deeper dive into how chatbots are revolutionizing customer service in the financial industry.
Challenges of Implementing LLMs in Finance and Banking
Large language models are truly revolutionizing the finance and banking domains. However, with all the opportunities, there come certain challenges that need to be navigated carefully.
Let us look at some of the obstacles the industry is facing while implementing large language models -
Navigating Regulatory Compliance -
The regulatory framework in the banking and finance industry is intricate and constantly evolving. Stringent regulations govern data privacy, security, and fair lending practices. LLMs, however, can sometimes struggle with transparency. Their decision-making processes can be difficult to trace and analyze, hindering adherence to regulations and raising concerns about explainability.
Further complicating matters are data protection laws like the GDPR (General Data Protection Regulation) in Europe. To comply, LLMs in finance and banking must be trained on specifically curated datasets, adding complexity to data management.
Addressing Data Privacy and Security Concerns -
Banks and financial institutions handle a vast amount of sensitive personal data. Implementing LLMs necessitates meticulous training on datasets specifically designed to prevent data breaches or unauthorized access.
Robust encryption and stringent access controls are crucial. However, striking a balance between security and maintaining the model's effectiveness can be challenging.
Managing Technology Updates and Maintenance -
LLMs in finance and banking should be updated with the latest technological progress to gain and maintain a competitive advantage over peers. But the process takes work. Each update requires rigorous testing. The goal is to protect the large language models against potential cyberattacks.
The finance industry is heavily regulated, so considering any technological update is difficult. Also, technological advancements do not affect the quality of the model or its performance.
Training and Upskilling Employees for LLM Usage -
Implementing large language models in daily operations can invite resistance from organizational employees. People have been used to establish workflows and procedures for years.
Additionally, the employees must understand and develop a deep knowledge of LLMs, and the ethical considerations involved in implementing them add to their complexity.
Ethical Considerations and Potential Solutions -
Beyond the challenges mentioned above, ethical considerations surrounding LLM use in finance require careful attention. Potential biases within the training data can lead to discriminatory outcomes. Additionally, the "black box" nature of some LLM models can make it difficult to understand their decision-making processes, raising concerns about fairness and accountability.
The good news is that there are ongoing efforts to mitigate these risks. Developments in explainable AI (XAI) techniques aim to make LLM decision-making more transparent. Additionally, creating robust training datasets that are diverse and unbiased is crucial.
So, will the potential of LLMs in finance and banking increase in the upcoming years? Let's find out.
Future Trends and Predictions of LLMs in Finance and Banking
The coming years hold immense promise for LLMs in finance and banking, with advancements in technologies like automated compliance and blockchain integration poised to reshape the industry. These innovations will not only mitigate errors but also significantly increase productivity and efficiency.
Let's delve deeper into some of these exciting trends:
Enhanced Customer Service -
Chatbots in the financial and banking sector are predicted to become significantly more intelligent in the coming years. A study by Juniper Research suggests that chatbot accuracy in finance could reach 85% by 2024, up from 72% in 2023. This translates to chatbots offering more relevant and tailored financial advice, along with a higher rate of successfully resolving customer inquiries.
The future of chatbots in finance extends beyond just accuracy. We can expect them to engage in more comprehensive conversations with a more human-like tone. Imagine a customer with limited financial knowledge seeking to open a credit card account. An advanced LLM-powered chatbot could seamlessly guide them through the process, answering questions and explaining terms in a clear and understandable manner.
Automated Compliance -
The future holds the promise of seamlessly interpreting and training LLMs while effortlessly applying regulatory frameworks. This will empower LLMs in finance and banking to automate time-consuming tasks like monitoring activities and updating policies. Robotic Process Automation (RPA) will play a crucial role in achieving this.
Automating compliance not only frees up valuable employee time for more strategic tasks but also significantly reduces errors that are prone to occur in traditional manual methods.
Integration with Blockchain -
Analyzing blockchain data enables LLMs to audit in real-time, helping the models adhere to the industry's compliance standards. In addition, LLMs in finance and banking obtain transaction histories with the help of blockchain and detect potential fraud.
In addition, LLMs will enhance the security of blockchain networks by analyzing patterns and identifying vulnerabilities that traditional methods will overlook. All these functions help improve the trustworthiness of implementing LLMs in the banking and finance industry.
Improved Financial Literacy -
LLMs in banking analyze a customer’s financial and transactional history. Based on this, the LLM offers personalized suggestions regarding savings, budgeting, resource utilization, and meeting economic needs.
There are many complex ideas in finance that people outside the domain cannot comprehend. LLMs in finance will help in breaking down these complex concepts into simpler terms and make people financially smarter.
Furthermore, LLMs can address the needs of specific demographics, for example, by suggesting improved retirement planning strategies for senior citizens.
Implementation of LLMs by HDFC Bank -
HDFC Bank, aiming to stay competitive, implemented LLMs via Gen AI services. A private LLM solution powers a customer website that analyzes data (spending habits, history) to provide personalized recommendations.
Steps:
- Data Integration: Customer behavior data (spending, history, interactions) was integrated.
- Beta Testing: The LLM-powered website launched in beta for initial feedback and improvement.
- Personalization & Automation: The website analyzes data and offers personalized advice, automating tasks for efficiency.
Results:
HDFC Bank reports increased customer satisfaction (faster service) and improved operational efficiency. This exemplifies LLMs' potential to transform customer interactions in banking.
How Can Signity Assist You?
As we've seen, LLMs are revolutionizing finance and banking. If you're considering LLM implementation for your financial services, Signity can help you get started. -
- Chatbot Assistance and Language Understanding
- Multilingual Translation and Language Generation
- AI-Powered Legal Analysis
- Content Creation for Virtual Influencers
- Automated Coding and Program Synthesis
From AI-powered legal analysis to multilingual customer support, Signity's expertise in Generative AI and LLM development unlocks a world of possibilities. Let Signity help you leverage these technologies to redefine your banking operations and elevate the customer experience.