MLOps vs AIOps – What’s the Difference?
MLOps oversees the complete life cycle of ML models, from development to deployment and production. In contrast, AIOps automate and streamline IT operational processes, reducing the time and effort needed for manual tasks. This blog offers in-depth insights into MLOps vs AIOps.
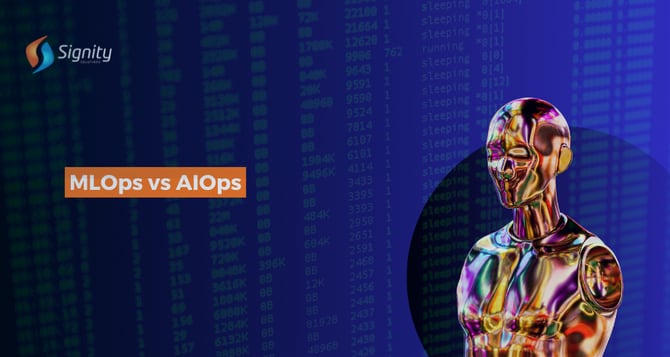
AI and ML consistently transform varied industries while providing advanced solutions quickly and efficiently. These technologies enable businesses to work smarter by automating tasks and making their business processes run smoother.
Machine learning operations (MLOps) specialize in managing the lifecycle of machine learning models from development to deployment and production. On the other hand, AIOps reduces time and effort by automating and optimizing IT operations processes.
This blog is aimed at providing complete insights on MLOps vs. AIOps and how it can help your businesses streamline their processes. Read on to learn more.
Key Takeaways
- Today's enterprises depend on AIOps and MLOps to support AI and ML environments. Each addresses different aspects while working together to meet ITOps needs.
- AIOps involves various analytics and AI initiatives to optimize IT operations, while MLOps focuses on the operational aspects of ML models, such as efficient deployment, monitoring, and maintenance.
- MLOps focuses on different data types for building predictive models, while AIOps deals with operational data from IT systems to detect patterns and anomalies.
- MLOps integrates with Kubernetes, Docker, and Git for model versioning, CI/CD, and containerization. AIOps uses AI algorithms, big data processing, and monitoring tools to analyze real-time IT operations data.
- MLOps focuses on continuous monitoring and performance tracking of machine learning models in production, while AIOps continuously monitors IT systems to identify and resolve issues autonomously.
Key differences between MLOps and AIOps
MLOPs and AIOps methodologies share some similarities because of their roots in AI. However, both technologies serve different purposes and operate in varied contexts. Take a quick glimpse of both technologies in our section below.
Parameter |
MLOps (Machine Learning Operations) |
AIOps (Artificial Intelligence for IT Operations) |
Focus Area |
ML model development, deployment, monitoring, and performance optimization |
IT operations, incident detection, root cause analysis, and automation |
Main Users |
Data scientists, ML engineers, DevOps teams |
IT operations teams, system administrators |
Key Technologies |
Python, TensorFlow, PyTorch, Jupyter, and Notebooks |
Machine learning, AI algorithms, Big Data, and monitoring tools. |
Key Components |
Data pipelines, model training, model deployment, and monitoring |
Data collection, AI-driven insights, automation of IT tasks |
Primary Objective |
Ensures the smooth operations of machine learning models |
Optimizes IT operations by predicting issues and automating tasks |
Impact on Business |
Enhances business insights, customer experiences, and decision-making through predictive analytics |
Improves IT reliability, reduces downtime, and minimizes operational costs |
Use Case |
Applied to machine learning (ML) and data science projects for predictive analytics and AI |
Applied in IT AI operations to enhance network, system, and infrastructure management. |
Industry Application |
Data-centric industries like finance, healthcare, retail |
IT-heavy industries like telecom, cloud services, and large enterprises |
Now that you have a quick glimpse of AIOps vs. MLOps, let's gain detailed insights into both technologies.
MLOps
MLOps is a technology that helps scale, build, and deploy multiple algorithms to maintain consistency in production. It also aims to enhance collaboration between the operations unit and data scientists within an organization.
It provides a multidisciplinary approach to managing ML algorithms in ongoing products throughout their lifecycle.
The idea of MLOps covers everything involved in the life of machine learning models, including preparing training data, developing, training, and deploying models, and infrastructure monitoring and maintaining models in production.
The above image shows how MLOps is used at each stage of the ML model lifecycle.
Key components of MLOps
Projects involving machine learning typically begin with defining a business use case. Once the use case is defined, the next steps include deploying the machine-learning solution into production.
- Data Extraction: Bringing in data from different sources
- Exploratory Data Analysis: Analyzing the data and its features
- Data Preparation: organizing data for a successful ML solution
- Developing ML Model/Solution: Building and training an ML model using ML techniques
- Model Evaluation and Validation: Testing the model with data to check its performance
- Model Deployment: Deploying ML models in production
Building and processing ML systems is a hands-on process, and it's not easy to manage such systems at scale. Many teams find it challenging to deploy and manage ML solutions manually. That is where MLOps help!
Using the MLOps best practices and a step-by-step approach, as mentioned above, can make building, deploying, managing, and even monitoring the ML systems a streamlined process.
Advantages of MLOps
MLOps is an advanced methodology focused on developing scalable machine learning systems. It offers businesses a wide range of benefits that help deliver project success.
Managing Machine Learning Lifecycle
An ML lifecycle is comprised of varied steps, and it can be challenging to organize and manage it all using traditional methods. MLOps offers a competitive edge by quickly optimizing and deploying machine learning models in production.
Multiple Pipeline Orchestration
ML model development involves a combination of varied pipelines, including pre-processing, model validation, and feature engineering models. It helps orchestrate these pipelines to update the model automatically.
Continuous Integration and Deployment
MLOps has a variety of tools and techniques, and CI/CD can be used to deploy the ML systems successfully.
Scale ML Apps
Since the app's data and usage increase with time, it can cause ML app failures. However, with MLOps, it is possible to scale the ML apps as business demand increases.
Monitor ML Systems
Monitoring ML systems is essential to ensure a smooth performance. Provides monitoring methods by enabling the identification of model and data drifts.
Better Governance and a Faster Validation Process
MLOps solutions produces automatic reports using a strict governance framework that allows for traceability.
Higher Innovation and Productivity
MLOps tools are made for data experts, IT professionals, and data teams. They offer easy-to-use systems with carefully selected data resources. This leads to faster development and deployment processes while speeding up workflow.
Monitorability
MLOps solutions continuously retrain the model to maintain accuracy and signal if there's a drop in performance. This ensures compliance with regulations and the delivery of high-quality information.
Faster time to Market
Businesses can speed up the development and deployment of new machine learning-powered products and services by using MLOps services to make the process more efficient.
Use Cases of MLOPs
Machine learning (ML) and data science projects for predictive analytics and AI.
Real-life use cases of MLOps
- Coinbase and AT&T employed MLOps for web analytics.
- Bloomberg leveraged the capabilities of MLOps for share market analysis.
- ESPN and Sky Sports used MLOps for performing sports analysis.
AIOps
According to Gartner, enterprise IT infrastructure produces an average of 2 to 3 times more IT operations data each year. Traditional IT management solutions may struggle to handle this large volume and resolve issues effectively.
In such a scenario, enterprises require an automated solution that can alert IT staff to significant risks, identify problems, and autonomously resolve repetitive issues instead of relying solely on staff manual monitoring.
AIOps uses big data and machine learning to automate IT operations. It helps with event correlation, anomaly detection, and causality determination.
AIOps replace tedious and manual operations with intelligent AI-driven solutions, following a quick step-by-step process, as shown in the image above. This helps identify patterns and events, collect structured and unstructured data, report issues, and reduce noise.
This greatly improves the team productivity, which is responsible for keeping the operations up and running.
Key components of AIOps
Since no one size fits all, every enterprise requires a unique AIOps solution. These AI solutions spot and react to real-time issues effectively. To do so, some of the core elements of AIOps contribute well. Below are some of the key components of AIOps.
Machine Learning
IT analytics is all about finding patterns. Machine learning allows AIOps to use the machine's computational power to identify these patterns in IT data.
Anomaly Detection
Any modifications in the system behavior can lead to a non-responsive system, poor customer experience, and increased downtime. With AIOps, businesses can quickly detect any type of unusual activity or behavior.
Predictive Insights
AIOps can incorporate predictive insights into IT operations. This can help IT staff be proactive in identifying issues before they occur and reduce the number of service desk tickets.
Automated Root Cause Analysis
Gaining insights alone is not sufficient. Businesses must also be able to take action. Traditional solutions are unable to manage and resolve issues on time, while AIOps identify the root cause in the background automatically, leading to quick issue resolution.
Benefits of AIOps
AIOps improve IT operations and provide a myriad of benefits to businesses looking to incorporate advanced solutions into their processes. Below are some of them.
Data-Driven Decision-Making
AIOPs leverage machine learning techniques in IT operations, such as historical data analysis, predictive analysis, and pattern matching. These techniques allow businesses to benefit from data-driven decisions while reducing human error. This automated data-driven insight allows IT operations to act on resolving the issue rather than focusing on identifying the root cause.
Proactive IT Operations
Delivering greater customer satisfaction is of the utmost importance to staying ahead in this competitive environment. Just responding to these issues alone is not sufficient; it is also essential to predict if any failure could occur. So, to ensure predictability, AIOps empowers the UT teams with the capability of predictive analysis that enables them to predict and resolve issues beforehand.
Detecting Anomalies
IT operations teams can identify unusual behavior by using ML techniques like clustering. AIOps help develop monitoring techniques that can be further used in anomaly detection.
Enhanced time allocation and priority setting: AIOps help handle large amounts of data. It separates valuable information from irrelevant data, adds important context to event data, and prioritizes it effectively.
Minimizing IT Costs
AIOps tools can help reduce costs by automating many IT operational tasks. They can identify and prevent potential issues before they cause expensive system outages. This proactive approach minimizes downtime, service disruptions, and financial losses.
Use Cases of AIOps
Real-life use cases of AIOps
- Place Park Technologies and TDC NetDesign used AIOps for predictive alerting.
- Schaeffler Group leveraged AIOps to avoid service disruptions.
- Enablis used AIOps to monitor the system properly.
Conclusion
In today's data-driven world, businesses can maintain a competitive edge by implementing AIOps and MLOps. When comparing MLOps and AIOps, it's important to note that they both involve using AI and machine learning, but they focus on different things.
MLOps is all about making machine learning work well and helping people use data to make better decisions. AIOps, on the other hand, is about managing IT systems and keeping them running smoothly.At Signity Solutions, we have extensive experience in AI and ML technologies and a team of skilled developers. We offer advanced solutions tailored to unique business needs.
Wondering if MLOps or AIOps suits your business requirements? Let's talk.
Frequently Asked Questions
Have a question in mind? We are here to answer. If you don’t see your question here, drop us a line at our contact page.
Is AIOps the same as MLOps?
No, MLOps focuses on the entire lifecycle management of machine learning models, including development, deployment, monitoring, and production. On the other hand, AIOps leverages ML and AI to automate and enhance IT operations, such as issue detection and resolution.
Do MLOps and AIOps work Together?
AIOps and MLOps are focused on different objectives, but they can complement each other. AIOps platforms may use ML models developed through MLOps processes to automate IT tasks and analyze operational data, improving overall system efficiency.
Which Industries Benefit From MLOps?
MLOps benefit industries that heavily rely on data-driven decision-making, such as finance, healthcare, retail, and tech. These industries can streamline their machine-learning workflows and optimize model performance.
What Types of Businesses Need AIOps?
Large-scale businesses with complex IT environments, such as telecom, cloud service providers, and e-commerce, can benefit from AIOps. With this, they can automate their IT operations, reduce downtime, and improve system reliability through AI-driven insights.
Which is more Suitable for Small Businesses: MLOps or AIOps?
The selection between MLOps and AIOps depends heavily on the business focus. Small businesses focused on developing machine learning models will benefit more from MLOps. On the other hand, small businesses with complex IT infrastructures might consider AIOps to streamline IT operations.