RAG in Financial Services
Integrating RAG in financial services can help your business in several ways. From protecting data to streamlining processes, this technology is a must. Explore RAG use cases, technical aspects, implementation practices, and deployment in the business.
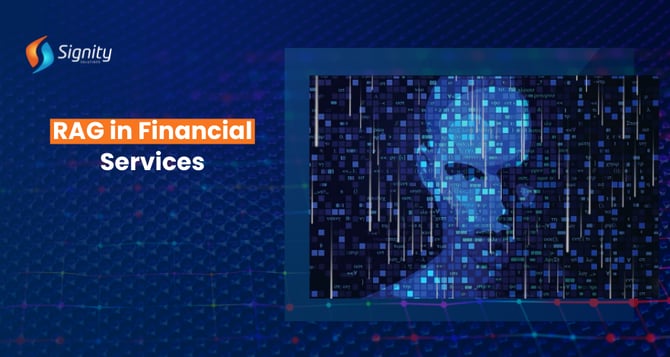
One of the major challenges in financial services is handling a large amount of data. It is crucial for businesses to protect the sensitive information of their customers securely in order to protect against any fraud.
It is where retrieval augmented generation services comes in. RAG in financial services helps improve operational efficiency while staying compliant with evolving regulations.
Understanding RAG in Financial Services
RAG (Retrieval-Augmented Generation) is reshaping the financial industry by improving processes like anti-money laundering, asset liability management, and credit risk assessment. It leverages embed and vector databases to integrate with data via APIs seamlessly.
With its ability to streamline data retrieval and generation, RAG drives innovation across financial services, bridging the gap between complex data and actionable insights. The image below shows the query process with and without RAG.
This blog will help you understand how RAG improves fraud detection in banking along with its benefits, best practices, applications, and technical aspects.
Key Takeaways
- RAG enhances fraud detection, compliance, and decision-making with advanced data retrieval and generation.
- RAG in financial services streamlines operations, reduces costs, and delivers smarter, data-driven insights.
- RAG improves credit risk assessment, compliance, and investment advisory processes.
- Built on NLP, vector databases, and secure APIs, RAG ensures accurate, context-driven solutions.
RAG Use Cases for FinTech Businesses
Implementing RAG strategy into business can help gain a competitive advantage through smarter decision-making and better product profitability.
Here is how you can build a business case for RAG:
Demonstrating ROI
By demonstrating how RAG technology reduces expenses and enhances financial results, you can demonstrate the quantifiable return on investment. It results in operational cost savings from integrating RAG, and better strategic choices and more accurate financial forecasts are made possible by the real-time data insights it offers.
RAG Proof-of-Concept
Conduct a proof-of-concept to assess how it affects operational effectiveness, compliance optimization, and decision-making procedures. During this phase, businesses can test RAG's capabilities in a controlled setting, learning how it might improve regulatory compliance accuracy, expedite decision-making, and streamline procedures.
Streamlining Operations
RAG can be used in financial services to automate processes, cut down on duplication, and boost overall productivity. RAG reduces manual intervention and speeds up reaction times to market changes by swiftly retrieving and integrating data from many sources.
Data-Driven Financial Transformation
Use RAG to capture real-time data insights that will help finance become more innovative and wiser. RAG gives financial professionals immediate access to extensive data sets, enabling them to optimize portfolios, make well-informed decisions, and more accurately forecast market trends.
Improving Product Profitability
By incorporating RAG into pricing models, client segmentation, and market analysis, you may increase profitability. RAG helps better match goods and services to consumer needs by rapidly analyzing consumer behavior and preferences.
Compliance Optimization
RAG's capacity to promptly obtain pertinent data and guarantee conformance to changing requirements can help address regulatory problems. Financial organizations may keep ahead of regulatory changes by promptly revising compliance procedures and risk management plans because of RAG's real-time data capabilities and API integration.
Competitive Advantage
Adopting state-of-the-art RAG technology for sophisticated decision-making and consumer involvement can establish your company as a leader. Financial services companies can create individualized customer experiences, optimize service delivery, and foresee market movements ahead of their competitors.
Must Read: Learn more about the use cases of RAG in various industries.
Technical Aspects of RAG
This section will help you examine the technical structure and mechanics of RAG, highlighting aspects like NLP, embeddings, and vector databases.
1. NLP-Driven Query Analysis
RAG leverages advanced natural language processing (NLP) techniques to analyze queries, ensuring a precise understanding of user intent for better data retrieval and contextual accuracy.
2. Embedding and Vector Databases
By using embedding and vector databases, RAG efficiently represents high-dimensional data as numerical vectors, enabling fast and accurate searches across large datasets.
3. Generative Language Model
At its core, RAG integrates a generative language model to create coherent responses by synthesizing information retrieved from independent data sources.
4. Contextual Similarity
RAG ensures contextually relevant results by using vector-based data retrieval techniques that measure the similarity between queries and stored data points.
5. Secure API-Based Integrations
RAG incorporates secure API-based integrations, allowing seamless access to and retrieval of information from diverse, independent systems while maintaining data privacy.
6. Modular Architecture for Scalability
Built with a modular architecture, RAG supports flexible and scalable implementation, enabling efficient handling of high-dimensional data and seamless integration of additional functionalities.
Must Read - Understanding RAG: Architecture, Techniques & Development
Best Practices for RAG Implementation
Implementing RAG effectively requires adherence to proven best practices that ensure security, compliance, and performance. These practices help maximize RAG's potential while maintaining data integrity and regulatory alignment.
GDPR Compliance
By adding strong anonymization layers to safeguard user data and preserve privacy, you can be sure that RAG implementation complies with GDPR. Review and update data handling protocols on a regular basis to comply with GDPR regulations, especially those pertaining to data access permissions and data minimization.
Data Integrity
Maintain the correctness and dependability of the data used in RAG systems by putting in place stringent data integrity controls, such as frequent audits and validation tests. To guarantee data consistency across many systems and sources, establish procedures for data reconciliation and correction.
End-to-End Encryption
To ensure that only authorized users can access and retrieve information, employ end-to-end encryption to safeguard sensitive data both during transmission and storage. To protect against changing cyber threats and weaknesses, evaluate encryption techniques on a regular basis.
Audit Logs
To ensure accountability and transparency in RAG procedures, use thorough audit logs to track and monitor data access. Set up automated notifications for anomalous activity so that possible security breaches and compliance problems can be addressed quickly.
Cybersecurity Protocols
Incorporate strong cybersecurity measures to protect RAG systems against cyberattacks, illegal access, and data breaches. To detect and reduce threats, perform vulnerability assessments and update systems often with the most recent security updates.
Economic Data Sources
Portfolio management concepts and economic data sources should be used to improve RAG's prediction power. Update these resources frequently to give current information and guarantee that RAG can adapt to changes in the economy.
Role-Based Access
To ensure that only those with the proper authorization can view or alter important data, implement role-based access (RBAC), which limits data access based on the user's function within the company. This procedure lowers the possibility of unwanted access and preserves data security.
Must Read: Check out the real-world examples of RAG and how it is helping businesses.
Deployment and Timeline for RAG
RAG technology implementation starts with discovery and scoping, during which competitive analysis is used to identify business objectives and data requirements.
After that, the emphasis switches to feature prioritization to guarantee alignment with organizational goals, data infrastructure setup, data integration, and API development.
Model training and customization are carried out to tailor RAG to particular use cases, utilizing platforms like Oracle for smooth interaction.
Real-time data capabilities are evaluated, audit and traceability procedures guarantee compliance, and pilot testing and evaluation aid in performance validation prior to full-scale deployment.
Scalability and user training are prioritized after deployment to improve uptake and usability and help businesses effectively utilize RAG solutions.
Transform Financial Processes With Tailored RAG Solutions
Ready to integrate RAG into your financial business? Connect with us today.
Get Custom RAG Solutions for Your Finance Business
Ensure data security, seamless integration, and personalized services with custom RAG solutions.
As an expert AI development company with years of experience, we will help you develop the right solution that aligns with your business goals. Get in touch with our professionals today to build solutions at a reasonable price.
Frequently Asked Questions
Have a question in mind? We are here to answer. If you don’t see your question here, drop us a line at our contact page.
What is RAG in Finance?
Retrieval-Augmented Generation (RAG) in finance is a system that combines the retrieval of relevant external information with language generation to produce accurate and contextually informed responses for various financial applications.
How does RAG Improve compliance in Financial Services?
What are the Primary use Cases of RAG in Financial Services?
Key use cases include credit risk assessment, anti-money laundering (AML) processes, investment advisory, portfolio management, and operational efficiency improvements through data-driven insights.
How does RAG handle Real-time data in Financial Operations?
RAG uses advanced retrieval engines and real-time data integration capabilities to provide up-to-date insights, enabling quicker and more informed decision-making.
What technical Components are Involved in RAG Implementation?
RAG implementation involves technologies such as embedding and vector databases, NLP-driven query analysis, secure API-based integrations, and generative language models for high-dimensional data handling and contextual similarity.