Top 15 Large Language Models in 2024
Today, we’ll look at one of the top large language models (LLMs) in the world, with the top pick GPT-4. Like us, if you are wondering who else made it to the top, explore the top 15 large language models of 2024 and compare their features, performance, and pricing.
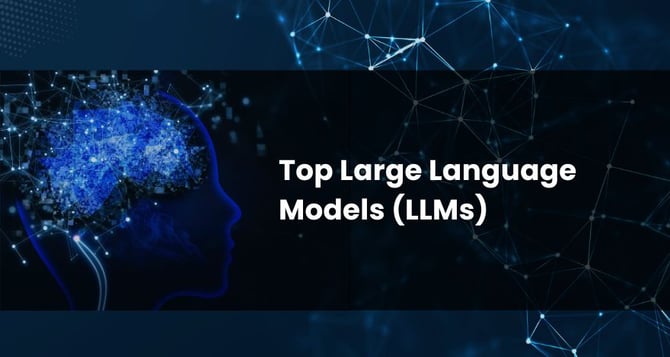
In the bustling realm of technology in 2024, it's an undeniable truth - one cannot skip the magnificent influence of "Generative AI" and the mighty Large Language Models (aka LLM) that fuel the intelligence of AI chatbots.
Especially after the rollout of ChatGPT by OpenAI, the pursuit to create the best LLM has taken a quantum jump. So, it would be right to say that many LLMs have been uncovered in a tornado of such competition.
Amid this dizzying crowd, the pressing questions that still persist -
- Which LLMs truly outperform in the race of being the most proficient?
- Which model is worthy of being crowned as the best LLM?
To shed some light, let's embark on a conclusive journey through the finest LLMs that are leading the charge in 2024.
Top 15 Large Language Models You Should Know in 2024
Investing in large language model development is increasingly crucial for companies aiming to maintain a competitive edge in the field of artificial intelligence (AI). It is not just a technological choice; it's a strategic imperative for companies seeking sustained growth, innovation, and competitiveness
Let's dive deeper into the wide-ranging array of some of the best Large Language Models that are the spearheaders in 2024. Plus, we offer a handpicked and unbiased compilation of LLMs, each extensively tailored to cater to distinctive purposes, opening new windows in the intricated world of AI.
So, let's step into the realism without putting on any airs.
1.) GPT 4 - The Fore-runner of AI Large Language Models
Rolled out to the public on 13 March 2023, this model has portrayed some really fantastic potential; it includes a deep comprehension of complicated reasoning, outperforms in several academic evaluations, has advanced coding skills, and demonstrates numerous other competencies that portray human-level performance.
Significantly, ChatGPT is the forerunner to integrate a multimodal capability, accepting both text and image inputs. Though ChatGPT hasn't handheld multimodal capability yet, some fortunate have already relished this exceptional multimodal via Bing Chat, which leverages the power of the GPT-4 model.
Furthermore, GPT-4 has substantially addressed and resolved the hallucination concern, making it a considerable leap in curating realism. In addition, when weighed against its predecessor, ChatGPT -3.5, ChatGPT-4 outweighs it by approximately scoring 80% of factual evaluations in different categories. OpenAI has poured huge efforts into employing Reinforcement Learning Human Feedback (RLHF) and domain-expert adversarial testing to achieve this proficiency.
In layman's terms, GPT-4 is not the conventional single, dense model we initially believed it to be!
Integrating GPT-4 is easily achievable through ChatGPT plugins. Regardless of its handful of challenges like higher inference time or slower response leading coders to opt for the GPT-3.5 model, the GPT-4 model stands unchallenged as the best Large Language Model available in 2024.
Transform your website into an interactive powerhouse! Discover How to Integrate ChatGPT into Your Website!
For applications, it's highly recommended to subscribe to ChatGPT, available for $20. On the same note, if you are looking for cost-free approaches, third-party portals offer access to ChatGPT-4 for free.
If you're ready to take your business to the next level, contact us today to learn more about creating custom ChatGPT development solutions.
2.) GPT 3.5 - A Speedy and Adaptable LLM
OpenAI's GPT-3.5 model is a general-purpose LLM that's actually hot on the heels of GPT-4. Though it didn't cover the specialized domain expertise of its sibling, it still excels in speed, generating complete responses within seconds.
Right from creative tasks like crafting essays to devising business plans, GPT-3.5 outperforms everything admirably. OpenAI has also extended the context length to a generous 16K for the GPT-3.5-turbo model. The icing on the cake is that it's free to use without any hourly or daily restrictions.
While GPT-3.5 is a powerful tool, it does have some shortcomings. It can be prone to hallucinations, sometimes generating incorrect information. This makes it a little less ideal for serious research work. But for basic coding queries, translation, comprehension of scientific concepts, and creative endeavors, GPT-3.5 makes a good investment.
In short, GPT-3.5 is a versatile and speedy LLM that's perfect for a wide range of tasks. Though it's not without its flaws, it's a great option for anyone looking for a powerful and affordable Large Language Model.
Want to implement Large Language Model solutions in your business?
Transform your business to new heights of success; get the best LLM development services from experts!
3.) PaLM 2 (Bison-001) - A Worthy Successor to PaLM
PaLM 2 is a large language model (LLM) developed by Google AI.
In simple terms, it is a successor to PaLM, which was launched in 2022, trained on a massive dataset of text and code, and can perform various tasks. Carving its own nook among the leading best large language models of 2024, we found Google's PaLM 2.
Google has enriched this model by focusing on different elements like commonsense reasoning, formal logic, mathematical equations, and advanced coding across a wide array of emerging over 20 languages. To your surprise, the most expansive iteration of PaLM 2 is reportedly trained on 540 billion parameters, gasconading a maximum contextual length of 4096 tokens.
To add, Google welcomed a quartet model solely depending on the PaLM 2 framework, in different sizes like Gecko, Otter, Bison, and Unicorn. Presently, Bison is the available offering.
In the MT-Bench test, Bison curated a score of 6.40, somewhat overshadowed by GPT -4's impressive 8.99 points. However, in reasoning evaluations, such as WinoGrande, StrategyQA, XCOPA, and similar tests, PaLM 2 exhibits an outstanding performance, even surpassing GPT-4. Its multilingual capabilities enable it to understand idioms, riddles, and nuanced texts from a multitude of languages – a feat other LLMs find challenging.
Ready to Explore More About Our Solutions?
Fuel your business with the brilliance of Large Language Models. Elevate your digital experience with our LLM development services.
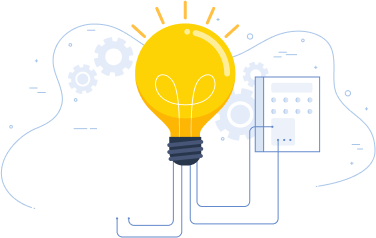
PaLM 2 also offers perks of quick responses, providing three at a time. Users can test the PaLM 2 (Bison-001) model on Google's Vertex AI platform. For customers to use, Google Bard, powered by PaLM 2, is the next one-stop solution.
4.) Bard - AI Assistant for Creativity, Learning & Discovery
Google Bard is an experimental conversational AI service powered by LaMDA (Language Model for Dialogue Applications) that Google AI is working on.
To your surprise, Bard, from the technical perspective, marks a subtle difference from other Large Language Models in a few ways. Firstly and foremost, it is designed to be conversational, meaning that it can carry on a dialogue with a user in a natural way, keeping it simple. Second, it is connected to the internet, which allows it to access and process information from the real world in real-time. This presents that Bard can offer more up-to-date and relevant information compared to other LLMs that are trained on static datasets.
All this training makes Bard capable of creating text, translating multiple languages, producing varied content, writing lines of code, and providing informational answers to questions asked.
BARD is among the leading multimodal large language models that help you tap into real-world data via Google Search. This facilitates it to understand and address a wider spectrum of prompts and queries.
5.) BERT (Bidirectional Encoder Representations from Transformers):
Developed by Google, BERT is a transformer-based language model known for its ability to understand context in natural language processing tasks. It has been widely adopted and has different variants, such as DistilBERT and RoBERTa.
Bert’s architecture has two primary parts: encoders and decoders. There are stacked encoders that creates meaningful results with given input. The output of the last encoder is then passed to inputs of all decoders for generating new information.
Source: towardsdatascience
BERT comes in two primary versions: base and large. Despite sharing an identical architecture, the key distinction lies in the number of parameters they employ. Specifically, BERT large boasts a substantial 3.09 times more parameters to fine-tune compared to its counterpart, BERT base.
6.) Cohere
Cohere stands out as an enterprise Language Model (LLM) designed for customizable training and fine-tuning tailored to the unique needs of a particular company. What sets Cohere apart is its flexibility in not being limited to a single cloud provider.
Cohere consists of two Large Language Models (LLMs), categorized into: generation and representation. Both types are available in a range of sizes, from small to xlarge.
7.) Azure Open AI Service - An Advanced Coding and Language Model
Azure Open AI services, rolled out by Microsoft, is a cloud-based platform that offers access to OpenAI's dynamic language models, consisting of GPT-3.5, Codex, DALL-E 2, and Whisper. Primarily, these models are an ideal choice to create and write different kinds of texts, translating language, and provide answers to your questions in an informative yet easy-to-understand way.
Not to forget, Azure OpenAI services provide tools to assist developers and data scientists in creating and deploying AI models quickly and easily.
In the simplest form, it's an advanced coding and language model. So, if you want to leverage large-scale, generative AI models with a comprehensive understanding of languages and coding to facilitate new reasoning and comprehension potential for developing cutting-edge apps, establish this coding and language model. Besides, you can detect and mitigate harmful repercussions with built-in responsible AI and evaluate enterprise-grade Azure security.
Beyond a shadow of a doubt, leverage generative models that are pre-trained with a trillion words.
The best part is to personalize generative models with labeled data for some specific scenarios by using REST API. Plus, fine-tune your model's hyperparameters to maximize the accuracy of outputs. Use some proven shortcut capabilities to offer the API with instances and accomplish the most relevant outcomes.
8.) Codex - Generating Code from Natural Language Description
Codex is a Large Language Model developed by OpenAI. It's a type of GPT (Generative Pre-Trained) model that's been trained on a huge amount of code and natural language data. And, considering the vast amount of data it's trained on, it can write code in several programming languages like Javascript, C++, Python, etc.
Adhering to the value it brings to technologically intensive organizations, it's a quantum leap with the potential to transform software engineering as a whole. Also, it can be used to build new AI-powered tools for code analysis and debugging.
9.) Claude V1 - ChatGPT Rival Right Now
Have you heard about Anthropic Claude, Google backs that?
It's a newbie on the Large Language Model block that's outperforming PaLM 2 in benchmark tests and offering a 100k token context window for the first time ever. This makes Claude a powerful tool for tasks like long-form text generation and code summarization with a motive to be helpful, honest, and harmless.
In multiple benchmark tests, Anthropic's Claude v1 and Instant models have shown great potential to deliver what it promises. In fact, Claude v1 performs better than PaLM 2 in MMLU and MT-Bench tests.
It's in bottleneck competition with GPT-4 and scored 7.94 in the MT-Bench test, while GPT-4 scored 8.99.
Anthropic Claude v1 scores 75.6 in the MMLU benchmark, while GPT-4 scores 86.4. However, Claude has a significant advantage in context window size, with 100,000 tokens compared to GPT -4's 10,000 tokens. This clearly means that Claude can process and comprehend much longer and more complicated prompts, making it an ideal choice for tasks like long-form text generation and code summarization.
Putting this in perspective, 100,000 tokens is equivalent to about 75,000 words. This means that you can easily load a full-length novel into Claude's context window, and remarkably, it would still understand it and create text in response to your prompts.
10.) Falcon - A Fresh Take on Open-Source AI
Fundamentally, the Falcon Large Language Model is a dynamic and scalable language model with remarkable performance and scalability. Besides, its open-source and freely available nature for commercial and research use makes it a valuable tool for researchers, developers, and businesses who want to harness the power of AI without the constraints of closed-source models.
Furthermore, Falcon LLM was trained on a colossal dataset of web text and curated sources, with custom tooling and a unique data pipeline to ensure the quality of the training data. This model also sheaths improvements like rotary positional embeddings and multi-query attention, which improves its performance. Its training took place on the AWS SageMaker platform with the help of a custom distributed training codebase, integrating 384 A100 40GB GPUs.
Falcon LLM is a decoder-only model:
Talking about its inescapable features, notably its "scalability." Its "multi-query attention" feature enables the model to manage large-scale tasks efficiently.
11.) LLaMa - A Foundational Model
LLaMa, aka Large Language Model Meta AI, is a branch of Large Language Models developed by Meta AI. The first version of LLaMa was launched in February 2023 and includes four model sizes - 7B, 13B, 33B, and 65B parameters (B stands for Billion).
Since it leaked, Meta's release of the LLaMA family of large language models (LLMs) has been a boon for the open-source community. As already told, LLaMA models are available in many sizes, from 7 billion to 65 billion parameters, and they have been shown to outperform other LLMs, such as GPT-3, on many benchmarks.
One of the unskippable advantages of LLaMA models is that they are open-source, meaning developers can easily fine-tune them and create new models to cater to specific tasks. This approach has led to rapid innovation in the open-source community, with new and improved LLM models being released regularly.
Another added feather in the hat is that LLaMA models are trained on publicly available data. This means that there are no potential red flags of proprietary datasets being used to train the models, which could potentially lead to bias or discrimination.
Meta's release of LLaMA models has been a sign of positive development for the open-source community. Eventually, it led to a rapid acceleration in innovation and the creation of new and improved LLM models that can be used to solve a wide range of problems.
12.) Vicuna - An Open-Source Chatbot with ChatGPT - 90% Quality
Introducing Vicuna 33B, an open-source chatbot and large Language Model created by a UC Berkeley, CMU, Stanford, and UC San Diego team, trained by fine-tuning LLaMA on user-shared conversations gathered from ShareGPT. It's an auto-regressive language model solely dependent on the transformer architecture.
Furthermore, the primary use case of Vicuna is research on LLM and chatbots, making intended users - researchers and hobbyists in Natural Language Processing, Artificial Intelligence, and Machine Learning. The best part was when preliminary evaluation showed Vicuna achieved more than 90% quality of OpenAI ChatGPT and Google Bard outperformed other models like LLaMA and Alpaca more than 90% of the time.
13.) Alpaca 7B - The Open Source ChatGPT Made for Less
ChatGPT got serious competition this time - not from Meta, Google, or even Baidu, but from Stanford University.
Stanford University claims to have developed a Generative AI chatbot that "acts qualitatively similarly to OpenAI's GPT-3.5" while being "exceptionally small and easy to create." Precisely, it costs less than $600 to be built.
The buzz is about Alpaca 7B, a fine-tuned form of Meta's seven billion-parameters LLaMA language model. This LLaMA model was fine-tuned using Hugging Face's training framework through "techniques like mixed precision and Fully Sharded Data Parallel training." Surprisingly, the 7b LLaMA model was fine-tuned in merely three hours on eight 80GB Nvidia A100 chips, costing less than $100 on cloud computing providers.
14.) Gopher - Deepmind - Revolutionizing The Future of Search Technology
Deepmind's language model "Gopher" is exact compared to the existing ultra-huge language models on numerous tasks, especially when answering questions about specialized subjects like humanities and science, or are nearly equal in other aspects, like mathematics and reasoning.
To your surprise, Gopher is smaller than some magnificently huge Large Language Models. Gopher sheaths nearly 280 billion parameters, or variables that can be tuned, eventually making it bigger than OpenAI's GPT-3.5 (having 175 billion parameters). But, it's smaller compared to Megatron, a collaboration system by Microsoft and Nvidia that has 535 billion parameters, as well as one developed by Google, with 1.6 trillion parameters, and also by Alibaba, having 10 trillion parameters.
At its core, its 7 billion parameters Retro model equals the performance of OpenAI's GPT-3. Also, researchers observed the exact section of training text used by the Retro software to create its output, making it easier to detect bias or misinformation.
15.) LaMDA
LaMDA (Language Model for Dialogue Applications) is developed by Google Brain announced in 2021. Powered by a decoder-only transformer language model, Lamda underwent pre-training on an extensive text corpus.
In 2022, LaMDA gained widespread attention when Google engineer Blake Lemoine went public with claims that the program was sentient. LaMDA is built on the Seq2Seq architecture.
These models’ sizes range from 2B to 137B parameters, LaMDA exhibits versatility by employing a single model to perform multiple tasks. It generates potential responses, which undergo safety assessments using external knowledge sources, ultimately being re-ranked to identify the most optimal response.
The Final Call
The headway these Large Language Models have made so far is merely the tip of the iceberg. Plus, the AI community's pursuit of overshadowing the benchmarks promises to make it more significant in the coming weeks, months, and years.
Precisely, in the not-so-far future, these 15 top Large Language Models will be indispensable tools in the Natural Language Processing domain. From the skillfulness of GPT-3 to the open-source approach of Falcon, there's no denying the way they revolutionized the present. These LLMs, with their revolutionary features, are reengineering the way we interact with natural technologies and creating new avenues for innovation.
Frequently Asked Questions
Have a question in mind? We are here to answer.
How do Large Language Models differ from traditional language processing approaches?
How can businesses integrate Large Language Models into their applications?
How do Large Language Models contribute to the future of artificial intelligence?