Types of Generative AI Models and Its Applications
If you are running your business without complete use of generative AI, you might fall behind. Know the types of generative AI models and their popular use cases to gain a better knowledge of how your brand can benefit from them, too.
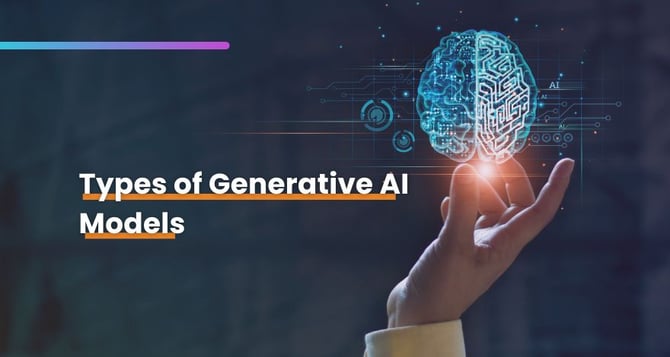
A generative AI model is a type of AI that can create new content, like text, images, and music, based on patterns and examples it has learned. It uses algorithms to generate original and realistic output without direct human input.
As reported by Forbes, in the next eight years, the value of generative AI will rise by $180 billion. With its most popular types like Variational Autoencoders (VAEs), Generative Adversarial Networks (GANs), and autoregressive models like the Transformer, generative AI ensures that companies across all industries can create better and faster content.
The use cases of generative AI are growing daily, making almost everything possible, from creating music to using AI based music visualizer solutions to creating top-quality AI video with little human intervention.
With our blog, we have explained the top six types of generative AI models and their use cases for several purposes. So, keep scrolling through to gain the best knowledge.
Key Takeaways
- To transform data analysis, problem-solving, and content creation, businesses need to implement generative AI.
- With major models VAEs and GANs at the forefront, generative AI is expected by Forbes to soar to $180 billion in eight years.
- 64% of CEOs understand how urgent it is to implement generative AI for improved company success. Marketers note improved performance, emphasizing the general acceptance of the benefits of generative AI.
- When incorporating generative AI, businesses must manage legal, financial, and reputational risks to ensure ethical and sustainable use.
Rising Industry of Generative AI
Before we begin with looking at the types and use cases, here are some numbers that you must look at to know how quickly this tech is growing, ensuring that adapting generative AI will be the best decision.
- The chart above shows that by 2032, the revenue in generative AI will reach 1.3 trillion.
- Among the entrepreneurs, 64% of executives have the sense of urgency to adopt generative AI models for better performance.
These numbers prove that entrepreneurs have realized the value of generative AI algorithms, and the majority have already started to make use of them. The others are planning to do so within the next two years.
Let us now look at the types of AI models that can be used for several purposes, such as creating content, images, videos, etc. One popular tool is an AI image generator, which can transform text prompts into unique visuals by leveraging deep learning techniques.
Want to know more about what is generative AI? Check our comprehensive guide.
Types of Generative AI Models
Generative AI models are a category of AI that holds the ability to create new and engaging data. There are several different types of generative AI models, each with its unique uses and features. Some of these include:
-
Generative Adversarial Networks (GANs)
Generative Adversarial Networks (GANs) are a class of generative AI models that consist of two neural networks-the generator and a discriminator. These are trained simultaneously through adversarial learning. The adversarial process enables the generator to produce increasingly realistic data.
GANs do not involve human intervention and enable applications in various domains, such as art generation, video enhancement, and data generation for training purposes. They are also widely used in areas such as image-to-image translation.
91.5% of Leading Businesses Invest in AI
Now is your turn to shine! Don't get left behind: Embrace AI and secure your business's future.
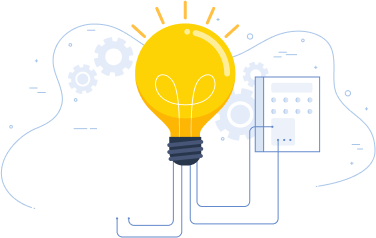
-
Variational Autoencoders (VAEs)
Variational Autoencoders (VAEs) are another type of generative model that creates new data by sampling from the learned latent space after encoding input data into a low-dimensional latent space. It then decodes it back to reconstruct the input. These models are applied to representation learning, data production, and compression problems.
These models are used for various tasks like data generation, compression, and representation learning.
-
Autoregressive Models
In autoregressive time series models, future values are predicted using past data from the same series and the assumption of a linear connection. The word "autoregressive" denotes that the variable is regressed on its historical values. These models employ lagged data of the relevant variable to generate predictions.
-
Transformer Models
Transformer models are a popular type of neural network architecture designed for subsequent data processing. These use a self-attention mechanism to determine the relative significance of words in a phrase for long-range interdependence and parallel processing of input sequences.
Transformer models have achieved state-of-the-art performance in several natural language processing tasks, like machine translation, text-to-speech, text generation, and sentiment analysis.
-
Deep Convolutional Generative Adversarial Networks (DCGANs)
Deep Convolutional Generative Adversarial Networks (DCGANs) are a class of deep learning models employed for generating synthetic images built on the foundation of convolutional neural networks (CNNs).
DCGANs have demonstrated exceptional capabilities in generating realistic images, paving the way for advancements in image synthesis and reconstruction tasks. Tools like the Depositphotos AI image generator can provide unique resources for creating high-quality visual content efficiently and effectively.
-
Recurrent Neural Networks (RNNs)
Recurrent Neural Networks (RNNs) are a class of neural networks designed for sequential data analysis. These contain loops that allow information to persist.
RNNs are well-suited for processing time series, speech recognition, and natural language processing tasks because of their ability to retain memory of previous inputs.
Future Outlook and Risks Associated with Generative AI
The development of generative AI models has the potential to revolutionize how we approach tasks like data processing, problem-solving, and text generation.
These AI models can also automate the process of converting text to PowerPoint presentations.
However, it also carries several concerns that need to be carefully considered.
It is essential to proactively address legal problems, such as those related to intellectual property and privacy, the monetary impact of the implementation and upkeep of new technologies, and reputational risks associated with their possible abuse or ethical dilemmas.
For generative AI to have a responsible and sustainable future, it will be imperative to strike a balance between utilizing its innovative potential and addressing its accompanying difficulties.
With a team of professionals at Signity, you can ensure that your business adapts applications of generative AI with the least risks. Our generative AI development services are designed to help brands drive the best results with the latest technologies. Get in touch with us today to know more.
Frequently Asked Questions
Have a question in mind? We are here to answer.
What are the 2 main types of generative AI?
What are different generative models?
Variational Autoencoders (VAEs), Generative Adversarial Networks (GANs), autoregressive models, and flow-based models are different generative models.
What is the most popular generative model?
The most popular generative model is the Generative Adversarial Network (GAN), which is widely used across several industries.
Is ChatGPT a generative AI model?