Enterprise RAG: Why Retrieval-Augmented Generation is Future of AI?
Retrieval-Augmented Generation (RAG) enhances enterprise AI by combining real-time data retrieval with language generation, providing accurate and context-aware responses. This blog examines how RAG works, its benefits, implementation tips, challenges, and why it is becoming a reliable choice for scalable enterprise AI solutions.
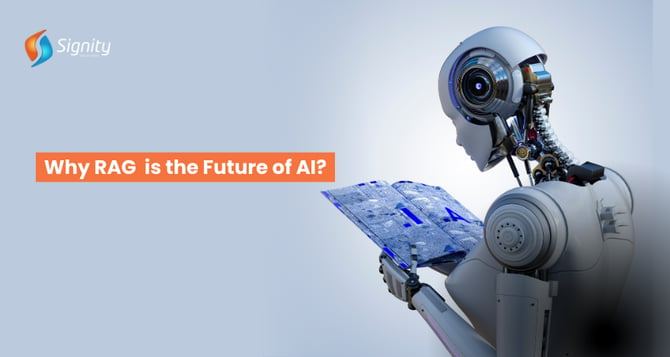
We're living in a time where artificial intelligence can draft emails, solve tickets, generate insights, and sometimes fabricate facts with the same confidence. Generative AI is brilliant, no doubt, but in the enterprise world, brilliance without reliability is a liability.
That's the turning point we've reached.
Retrieval augmented Generation solutions are a quiet change that's making Enterprise AI not just faster or smarter, but reliable and context-aware. RAG equips AI with real-time access to enterprise data, so it no longer generates responses from thin air; instead, it grounds them in your systems, documents, and data.
In this blog, we'll explore why Retrieval-augmented Generation is more than just a technical enhancement. It's the future blueprint for enterprise-ready AI.
We'll learn how it works, why it matters, and how companies are already using it to build a system that doesn't just answer a query, but one that provides insight and understanding.


- RAG enables AI to access trusted sources such as internal documents, product manuals, or knowledge bases, ensuring it provides answers grounded in your business context.
- Training or fine-tuning large models can be expensive and time-consuming. RAG offers a more flexible approach to obtaining domain-specific responses, eliminating the need to start from scratch every time.
- With the right data connected, a single RAG-enabled AI system can handle everything from customer support to legal queries to product troubleshooting, adapting to different teams and needs without extra overhead.
Understanding Retrieval Augmented Generation(RAG)?
Imagine suddenly being asked a question you are not familiar with. So, you quickly searched for it on Google, read top sources, and answered the questions with confidence and reliable information. This is exactly how Retrieval Augmented Generation works.
Implementing RAG is just like providing your AI with a highly accurate research center. RAG enables the AI system to search for information in real-time from a reliable and trusted source, rather than relying solely on the data it was trained on.
For an enterprise, RAG can be a beneficial tool. RAG enables your AI tool to extract knowledge from credible sources, including internal documents, wikis, product catalogs, and articles, to provide context-aware and accurate answers.
How is the RAG System Beneficial for Enterprises?
RAG enables businesses to modify generative AI models for domain-specific use cases without paying significant retraining expenses. Companies can utilize RAG to fill in the knowledge gaps in a machine learning model so that it can produce more accurate results.
Cost-effective Implementation and Scaling
Training a foundation model from scratch or even fine-tuning it with a smaller, industry-specific dataset can become a drain on both time and infrastructure. These processes require reworking the model's internal parameters to reflect better the new and specialized data, which often translates to high costs and considerable computing power.
RAG offers a smarter path forward. Instead of modifying the model itself, RAG equips it to tap into an organization's internal knowledge, secure documents, and real-time data. The result is improved performance and domain relevance, all without the overhead of retraining.
It's a scalable approach for enterprises to amplify the impact of AI while maintaining operational efficiency and the technical simplicity of such systems.
More Extensive Use Cases
The more relevant information a model has access to, the more versatile it becomes. When enterprises enrich a model's available knowledge, especially with internal or domain-specific data, they unlock its ability to respond accurately across a wider variety of use cases and business contexts.
RAG takes this further by enabling generative models to explore multiple data sources on demand. Whether answering layered queries or navigating highly specific subject areas, Retrieval-augmented generation (RAG) empowers models to retrieve relevant information from across systems in real-time, making them more adaptable, reliable, and scalable across different departments and workflows.
Enhanced Data Security
Unlike traditional fine-tuning approaches, where data becomes part of the model itself, RAG introduces a flexible bridge between the model and the information it needs. The model doesn't store internal enterprise data; instead, it references it when needed.
RAG allows organizations to retain full ownership and control over their first-party data, offering models read-only access that can be adjusted or withdrawn at any time. It's a secure, modular approach that supports dynamic data usage without compromising data governance.
Domain-Specific and Up-to-date Response
Generative AI models are only up-to-date with their last training data. After passing the cut-off point, their understanding of new developments begins to fade, making them less useful in evolving or specialized domains.
RAG addresses this limitation by bridging the gap between static model knowledge and real-time information. It allows enterprises to dynamically feed models with fresh, context-specific data, whether it's proprietary customer insights, internal documentation, or the latest research.
RAG ensures responses remain relevant, accurate, and grounded in the most up-to-date sources without requiring constant retraining.
Reduced AI Hallucinations
Large language models, such as GPT, generate responses by identifying statistical patterns in vast datasets. However, when no clear information is available or the model misreads context, it may fabricate answers that appear reliable but are actually false. This situation is known as AI hallucination.
Retrieval-Augmented Generation reduces this risk by shifting the model's dependency away from guesswork and toward verifiable information. Instead of relying solely on the training data, RAG frameworks allow models to reference curated, real-time, domain-specific, and new data.
This AI grounding leads to responses that are more aligned with facts and less prone to misinformation. While not a complete safeguard, it adds a critical layer of accountability and contextual precision to AI outputs.
Transform Your AI Strategy with RAG
Unlock the potential of real-time, data-driven responses and elevate your AI capabilities.
Key Considerations While Implementing RAG in Enterprise Processes
Bringing RAG into your enterprise is a thoughtful approach; it combines your company's knowledge, the AI's brainpower, and a robust system to help both work in sync. If you want it to work well and scale, here are four big areas you'll want to focus on:
1. Data Infrastructure
Before the model can do anything smart, it needs some smart data to work with. That means organizing your internal knowledge into accessible, clean, and consistently stored formats is important.
Whether you store your data in Google Cloud Storage, internal wikis, or CRM systems, ensure it is well-structured and easily accessible. You don't need to start everything at once; begin with what's most important, such as support documents, legal guidelines, or customer data, and then proceed with other tasks.
2. Retrieval Mechanisms
RAG is about understanding what someone is asking and surfacing content that matches the intent, not just the keywords search. That's where semantic search and vector databases come in. Tools like Pinecone or Vertex AI Matching Engine allow your system to fetch data that's actually relevant, even if the phrase doesn't match exactly.
This is the difference between a simple chatbot that says "I don't understand" and one powered with RAG that sounds like it is actually working at your company.
3. Integrating Model
The primary goal is to connect your retrieval system to your generative model in a way that feels seamless to the end user. You want the AI to read retrieved content like a quick briefing before answering, and not just deliver whatever it learned from training data.
This requires some orchestration, including deciding what data to send, how to format it, and how to handle token limits and latency. Tools like LangChain or LlamaIndex can help integrate these things, or you can build your logic.
4. Evaluation Metrics
RAG changes how we evaluate AI performance. It's not just about how well the model works. It's about providing the right information, staying accurate, and responding quickly.
Here are some important things to track:
- Are we retrieving the relevant documents for the query?
- Are the generated responses providing contextual information?
- Is the response time fast enough for a good user experience?
- Is the AI generating facts or confidently making things up?
Challenges For RAG Systems
RAG represents a significant advancement in enterprise AI, but it is not without its complexities. While it bridges the gap between language models and live knowledge, it also introduces several familiar challenges and some new ones. Before you think about deploying RAG across your organization, let’s break down the real-world challenges that come with the territory.
1. Limited Multi-Step Reasoning
While RAG systems can deliver impressive answers at the surface level, they often fall short when faced with complex, multi-layered tasks. Their ability to simulate reasoning is still bounded by deterministic logic, lacking the nuanced, contextual judgment that humans apply in iterative decision-making.
As a result, responses to complex queries may fail to capture the depth or subtlety required.
2. Language Sensitivity and Misinterpretation
Human language is contextual and often ambiguous, a quality that RAG systems are still learning to handle. Despite advances in semantic search, subtle shifts in phrasing can mislead the model, resulting in responses that fail to capture the intent.
While RAG reduces hallucinations more effectively than traditional LLMs, it does not eliminate them altogether.
3. Infrastructure Demands and Cost Overhead
RAG introduces architectural complexity. Fast and relevant retrieval depends on high-performance vector databases, efficient indexing, and powerful backend compute.
Supporting real-time, low-latency retrieval and response generation requires significant investment in cloud infrastructure, GPUs, and network bandwidth, making deployment cost-intensive and operationally demanding.
4. Dependency on Data Quality
RAG is only as good as the data it can access. If the source content is biased, outdated, or poorly structured, those issues directly impact the model’s output. Inconsistent, low-quality, unstructured data and documents can create knowledge gaps, limiting the value RAG is meant to add.
Ensuring clean, current, and diverse datasets is non-negotiable.
5. Responsible Deployment is Not Optional
Deploying RAG responsibly requires more than just technical integration; it also necessitates effective management and oversight. It’s about establishing an ethical framework that governs the use of data, the attribution of content, and the enforcement of human validation.
Enterprises must be deliberate in curating datasets, crediting original creators, and embedding review mechanisms into every RAG-driven workflow.
Conclusion
RAG is a shift in how we build intelligence into enterprise systems, striking a balance between performance, trust, and creativity with control. For organizations ready to scale AI responsibly, RAG offers not just a smarter way forward but a more grounded one. The future of enterprise AI will be built on retrieval, not on guesswork.
Take Your AI to the Next Level With RAG
Discover how RAG can transform your enterprise AI systems into accurate, real-time decision-makers.
If you are looking for an excellent AI development company, contact Signity Solutions. We help enterprises design and implement AI powered by RAG and other cutting-edge AI frameworks. If your organization is exploring the next phase of AI adoption, RAG offers a strong and future-ready foundation, and Signity can help you get there with confidence.
To begin creating intelligent, scalable, and future-ready solutions, contact our AI experts.