Google Creates Tx-LLM for Drug Discovery
Google has introduced Tx-LLM, a specialized large language model for drug discovery. Tx-LLM, trained on extensive biomedical data, predicts molecular interactions, screens compounds, and suggests new drug applications. It offers API access, custom solutions, and consulting services. This AI-driven tool aims to make drug development faster, more efficient, and successful.
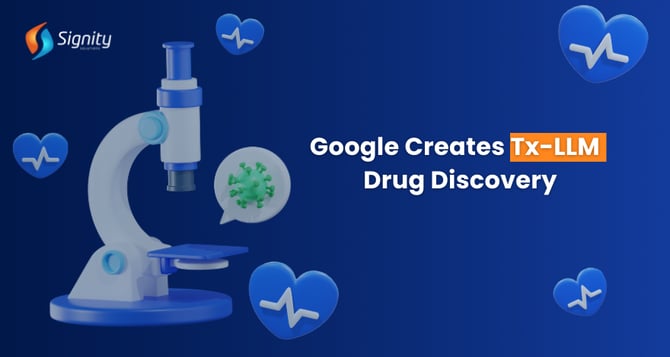
In a groundbreaking development in the field of pharmaceuticals, Google has unveiled its new large language model, Tx-LLM, designed specifically for drug discovery. This innovation marks a significant leap forward in the application of artificial intelligence (AI) to one of the most complex and critical areas of medical research.
Background and Motivation
The pharmaceutical industry faces numerous challenges, particularly in the drug discovery phase, which is often lengthy, costly, and fraught with high failure rates. Traditional methods rely heavily on extensive laboratory work, clinical trials, and a degree of trial and error.
In recent years, AI and LLM development services have shown promise in streamlining this process, offering new ways to predict how drugs will interact with targets, optimize drug formulations, and identify potential new uses for existing medications.
Google, leveraging its expertise in AI and machine learning, has entered this arena with Tx-LLM, a tool that aims to revolutionize how researchers approach drug discovery.
What is Tx-LLM?
Tx-LLM stands for "Therapeutics Large Language Model." It is a specialized version of the large language models (LLMs) that Google has been developing, tailored specifically for the healthcare and pharmaceutical sectors. These models are built upon the architecture of advanced neural networks, capable of understanding and generating human-like text based on vast datasets.
LLM Development
The development of LLMs, including Tx-LLM, involves several sophisticated steps:
Data Collection
Gathering vast amounts of text data from diverse sources, including scientific literature, clinical trial databases, and chemical compound libraries. For Tx-LLM, this also includes specific biomedical datasets.
Training the Model
Using powerful computational resources to train the model. This involves running complex algorithms that adjust the neural network based on the input data, allowing the model to learn language patterns and specific knowledge areas.
Fine-Tuning
Once the base model is trained, it undergoes fine-tuning on domain-specific data to improve its performance in targeted applications. For Tx-LLM, this means extensive training in biomedical and pharmaceutical data.
Validation and Testing
Ensuring the model's accuracy and reliability through rigorous validation and testing processes. This step is crucial to ensure that the predictions and insights generated by Tx-LLM are scientifically sound and useful for researchers.
Key Features of Tx-LLM
Extensive Training on Biomedical Data
Tx-LLM has been trained on a diverse and comprehensive dataset encompassing biomedical literature, clinical trial results, chemical compound databases, and molecular biology texts.
This allows it to have a deep understanding of the complex language and concepts in the field of drug discovery. Additionally, Tx-LLM benefits from Google's LLM services, providing extensive support and customization options for researchers.
Predictive Capabilities
One of the most potent features of Tx-LLM is its ability to predict molecular interactions and potential drug efficacy. By analyzing data patterns, Tx-LLM can suggest which compounds might interact favorably with specific biological targets, significantly accelerating the initial stages of drug development.
Natural Language Processing
Tx-LLM excels in natural language processing (NLP), enabling it to read and understand vast amounts of scientific literature. It can summarize research papers, extract key findings, and provide insights that would take human researchers much longer to uncover.
Enhanced Collaboration
Tx-LLM facilitates collaboration between different research teams and disciplines. By providing a common platform for data analysis and interpretation, it helps integrate diverse insights, fostering a more holistic approach to drug discovery.
LLM Services
Google’s initiative goes beyond developing Tx-LLM; it encompasses a suite of services designed to integrate and support the model's use in practical applications:
API Access
Providing access to Tx-LLM through APIs, allows researchers and developers to integrate its capabilities into their own tools and platforms seamlessly.
Custom Solutions
Offering customized versions of Tx-LLM tailored to the specific needs of different pharmaceutical companies or research institutions, ensuring that the model meets their unique requirements.
Consulting and Support
Google offers expert consulting services to help organizations implement and optimize the use of Tx-LLM, providing technical support and guidance throughout the deployment process.
Continuous Updates
Regularly updating Tx-LLM with new data and improved algorithms to ensure it remains at the cutting edge of drug discovery technology.
Applications in Drug Discovery
Target Identification
Tx-LLM can assist researchers in identifying potential biological targets for new drugs by sifting through genomic and proteomic data.
Compound Screening
It can rapidly screen large libraries of chemical compounds to identify those most likely to have the desired therapeutic effect.
Drug Repurposing
By analyzing existing drugs, Tx-LLM can suggest new therapeutic applications, potentially accelerating the development of treatments for conditions that currently have limited options.
Clinical Trial Optimization
Tx-LLM can help design more effective clinical trials by predicting outcomes and identifying optimal patient populations.
Challenges and Future Directions
While Tx-LLM represents a significant advancement, there are still challenges to be addressed. Ensuring the accuracy of predictions, managing the vast amounts of data involved, and integrating Tx-LLM seamlessly into existing workflows are all areas that require ongoing work. Additionally, ethical considerations, such as data privacy and the implications of AI-driven decisions in healthcare, must be carefully managed.
Looking forward, Google plans to continue refining Tx-LLM, incorporating feedback from early adopters and expanding its capabilities. There is also potential for Tx-LLM to be integrated with other emerging technologies, such as quantum computing and advanced robotics, to enhance its effectiveness further.
Conclusion
Google's Tx-LLM stands at the forefront of AI-driven drug discovery, offering a powerful new tool for researchers in the pharmaceutical industry. By harnessing the power of advanced language models and LLM services, Tx-LLM promises to make drug discovery faster, more efficient, and potentially more successful.
As this technology evolves, it holds the promise of bringing new treatments to market more quickly, ultimately benefiting patients worldwide.