8 End-to-End MLOps Platforms You Must Try in 2024
Discover leading MLOps platforms for 2024, offering robust capabilities in data management, model training, deployment, and monitoring. Choose the ideal platform to optimize your machine learning workflows and achieve business objectives.
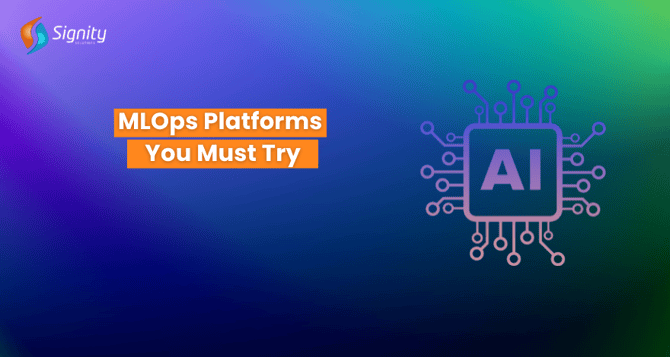
Machine Learning Operations (MLOps) is revolutionizing the way organizations develop, deploy, and manage machine learning models. An effective MLOps platform can streamline the entire machine learning lifecycle, from data preprocessing and model training to deployment and monitoring. Here are eight end-to-end MLOps platforms that you must try to enhance your ML workflows and drive business success.
Kubeflow
Kubeflow is an open-source MLOps platform designed to make machine learning workflows on Kubernetes simple, portable, and scalable. It provides a comprehensive suite of tools to manage ML pipelines, including Jupyter notebooks, TensorFlow model training, hyperparameter tuning, and model serving.
Key Features:
- Easy deployment on Kubernetes
- Supports various ML frameworks
- Scalable and portable workflows
MLflow
Developed by Databricks, MLflow is an open-source platform that manages the ML lifecycle, including experimentation, reproducibility, and deployment.
MLflow comprises four components: Tracking, Projects, Models, and Registry, each addressing a specific stage of the ML process.
Key Features:
- Experiment tracking and management
- Model packaging and deployment
- Centralized model registry
Tecton
Tecton is a feature store for machine learning that enables the creation, management, and serving of machine learning features. It simplifies the feature engineering process, ensuring consistency and reusability of features across different models.
Key Features:
- Real-time feature serving
- Automated feature engineering
- Integration with popular ML frameworks
DataRobot
DataRobot is an enterprise AI platform that accelerates the development and deployment of machine learning models. It offers automated machine learning (AutoML), model monitoring, and deployment capabilities, making it a powerful tool for data scientists and business analysts alike.
Key Features:
- AutoML for rapid model development
- Deployment and monitoring tools
- Collaboration and governance features
SageMaker
Amazon SageMaker is a fully managed MLOps platform by AWS that provides every developer and data scientist with the ability to build, train, and deploy machine learning models quickly. SageMaker offers a broad range of capabilities, from data labeling to model monitoring and retraining.
Key Features:
- Comprehensive suite of ML tools
- Integration with AWS ecosystem
- Scalable infrastructure
Azure ML
Azure Machine Learning by Microsoft is a cloud-based service that helps data scientists and developers build, train, and deploy models. It supports a wide range of ML frameworks and tools and offers robust MLOps capabilities for managing the end-to-end ML lifecycle.
Key Features:
- Integration with Azure services
- Automated ML and data labeling
- End-to-end ML lifecycle management
Google AI Platform
Google AI Platform is a managed service that lets you build, deploy, and scale ML models on Google Cloud. It integrates with other Google Cloud services, providing a seamless experience for data scientists and ML engineers.
Key Features:
- Support for TensorFlow, PyTorch, and other frameworks
- Hyperparameter tuning and training
- Scalable deployment and monitoring
H2O.ai
H2O.ai offers an open-source platform and an enterprise AI platform that provide a variety of tools for building and deploying machine learning models. H2O Driverless AI automates many aspects of the ML process, from feature engineering to model tuning.
Key Features:
- AutoML and driverless AI capabilities
- Wide range of ML algorithms
- Integration with big data platforms
Conclusion
Choosing the right MLOps platform can significantly enhance your machine learning projects. These eight platforms offer robust features to manage the entire ML lifecycle, from data preprocessing and model training to deployment and monitoring.
To maximize their benefits, consider engaging with MLOps consulting services and solutions for expert guidance and support. Try these platforms to find the best fit for your ML operations and drive business success.