The Role of AI in Predictive Maintenance
This article delves into AI's crucial role in Predictive Maintenance, predicting equipment failures, optimizing schedules, and transforming traditional approaches into proactive, data-driven strategies. AI enhances efficiency, reduces costs, and boosts reliability, fostering sustainable practices in various industries.
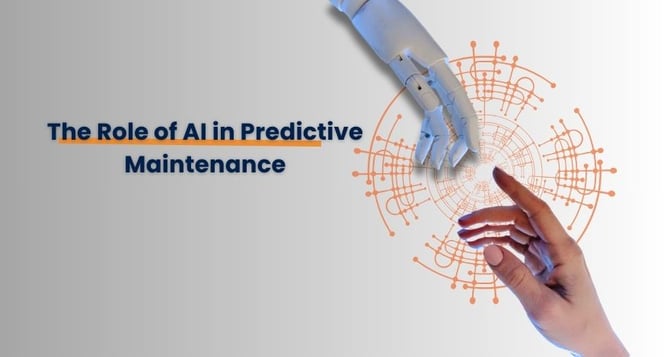
In today's fast-paced and competitive manufacturing landscape, unplanned downtime can be a costly disruption, leading to production delays, revenue losses, and reputational damage.
Manufacturers tackle challenges with AI-driven predictive maintenance (PdM). They optimize assets and reduce downtime using AI development services. This strategic approach positions businesses for success in industrial maintenance's evolving landscape.
Traditional Maintenance Strategies
Traditional maintenance strategies, often reactive, rely on scheduled maintenance intervals or on-site inspections to identify and rectify equipment failures. However, these methods can be inefficient and ineffective, as they often fail to detect early signs of impending failures, leading to unexpected downtime and costly repairs.
What is AI-Driven Predictive Maintenance (PdM)
AI-driven PdM offers a paradigm shift in maintenance practices, leveraging the power of machine learning and artificial intelligence to analyze vast amounts of data from sensors embedded in machinery. By analyzing patterns and anomalies in sensor data, AI algorithms can predict when equipment is likely to fail, allowing for proactive maintenance interventions before failures occur.
Benefits of AI-Driven PdM for Manufacturing
The adoption of AI-driven PdM in manufacturing brings a multitude of benefits, including:
Reduced Downtime: AI-driven PdM enables manufacturers to identify potential equipment failures early on, preventing them from escalating into catastrophic breakdowns. This proactive approach can significantly reduce unplanned downtime, ensuring uninterrupted production and improved operational efficiency.
Enhanced Asset Performance: By monitoring equipment health in real-time, AI-driven PdM can optimize asset utilization and extend asset lifespan. This proactive maintenance approach helps prevent premature equipment wear and tear, reducing maintenance costs and enhancing overall asset performance.
Improved Safety: Unplanned equipment failures can pose safety hazards for workers and the environment. AI-driven PdM can mitigate these risks by identifying potential failures before they occur, allowing for timely interventions and preventing hazardous incidents.
Optimized Resource Allocation: AI-driven PdM can provide valuable insights into equipment performance, enabling maintenance teams to prioritize tasks and allocate resources more effectively. This optimization leads to more efficient maintenance operations and reduced maintenance costs.
Data-Driven Decision Making: AI-driven PdM generates a wealth of data that can inform strategic decision-making. By analyzing historical and real-time data, manufacturers can gain insights into equipment behavior, identify areas for improvement, and make informed decisions regarding equipment upgrades or replacements.
How AI-Driven PdM Works
The process of AI-driven PdM involves several steps:
1. Data Collection: Sensors embedded in machinery collect data on various parameters, such as vibration, temperature, pressure, and electrical current.
2. Data Preprocessing: The collected data is preprocessed to clean and prepare it for analysis. This may involve removing noise, handling missing values, and transforming the data into a format suitable for machine learning algorithms.
3. Feature Engineering: Relevant features are extracted from the preprocessed data. These features should be informative and help distinguish between normal and abnormal equipment conditions.
4. Model Training: Machine learning models are trained using the extracted features and labeled data. Labeled data indicates whether the equipment is in a normal or abnormal condition.
5. Model Deployment: The trained models are deployed in the PdM system to analyze real-time data and generate predictions about equipment health.
6. Monitoring and Alerting: The PdM system continuously monitors equipment health and generates alerts when potential failures are detected.
7. Maintenance Intervention: Maintenance personnel are notified of the alerts and take corrective actions to prevent equipment failures.
Real-World Applications of AI-Driven PdM
AI-driven PdM is already making a significant impact in various manufacturing industries, including:
Power Generation: AI-driven PdM is used to monitor turbines, transformers, and other critical equipment in power plants, predicting potential failures and preventing unplanned outages.
Oil and Gas: AI-driven PdM is employed to monitor pipelines, pumps, and compressors in oil and gas operations, identifying potential leaks and malfunctions to prevent safety hazards and environmental damage.
Automotive Manufacturing: AI-driven PdM is utilized to monitor robots, assembly lines, and other manufacturing equipment in automotive plants, predicting potential failures and preventing production delays.
Aerospace: AI-driven PdM is applied to monitor aircraft engines, avionics systems, and other critical components in aerospace manufacturing, ensuring safety and reliability.
The Future of AI-Driven PdM
AI-driven PdM is rapidly evolving and is poised to play an even more transformative role in manufacturing. As AI algorithms become more sophisticated and as data availability increases, AI-driven PdM will become increasingly accurate and predictive, enabling manufacturers to achieve near-zero downtime and optimize asset performance for a competitive edge.
Technical Considerations for Implementing AI-Driven PdM:
Effective implementation of AI-driven PdM requires careful consideration of technical aspects, including:
Data Quality: The quality of sensor data is crucial for accurate predictions. Data cleansing and preprocessing are essential to ensure the integrity and reliability of data.
Model Selection: Choosing the appropriate machine learning algorithm for the specific equipment and failure modes is critical for effective prediction.
Model Training and Validation: Rigorous training and validation of machine learning models are necessary to ensure their accuracy and generalizability to real-world scenarios.
Continuous Monitoring and Improvement: AI-driven PdM is an ongoing process that requires continuous monitoring of model performance and data quality to adapt to changing conditions and equipment behavior.
Integration of AI-Driven PdM into Manufacturing Systems
The successful integration of AI-driven PdM into manufacturing systems involves:
Data Integration: Integrating sensor data with existing manufacturing data sources, such as enterprise resource planning (ERP) and manufacturing execution systems (MES), provides a comprehensive view of equipment health and operational performance.
Workflow Integration: Integrating AI-driven PdM predictions into maintenance workflows enables proactive scheduling of maintenance tasks, optimizing resource allocation, and minimizing disruptions to production.
Human-AI Collaboration: AI-driven PdM should complement, not replace, the expertise of maintenance technicians. Humans provide valuable insights and context to AI predictions, ensuring informed decision-making.
Change Management: Implementing AI-driven PdM requires cultural change within the manufacturing organization. Training and communication are essential to ensure the adoption and acceptance of AI-driven technologies.
Conclusion
AI-driven PdM is revolutionizing manufacturing maintenance practices, enabling manufacturers to achieve unprecedented levels of asset performance and operational efficiency.
Empower Your Innovation Journey with AI
From concept to deployment, we bring your vision to life through advanced AI development. Reach out to our experts to discuss your project!
By leveraging the power of AI to predict equipment failures and optimize maintenance schedules, manufacturers can minimize downtime, reduce costs, and enhance safety. As AI technology continues to advance, AI-driven PdM is poised to become an indispensable tool for manufacturing success in the years to come.