What is Data Annotation? Why You Need It
Data annotation is the process of labeling data to train AI models. It's essential for AI development services, enabling accurate predictions and real-world applications. Despite challenges like cost and time, it's critical for AI consulting companies and generative AI development services to achieve innovation and reliability.
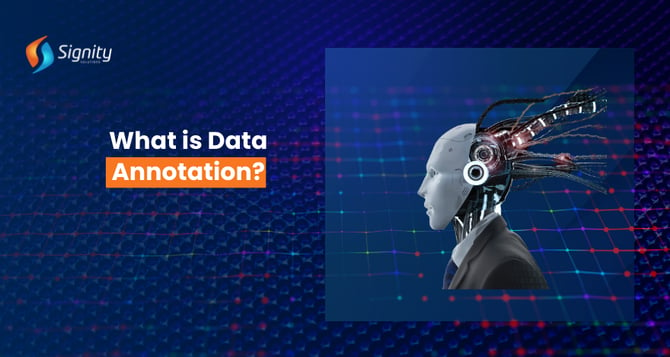
In today's data-driven world, the ability to leverage massive amounts of information is crucial for advancements in artificial intelligence (AI) and machine learning (ML). One of the fundamental processes that enable machines to learn from data is known as data annotation.
Data annotation involves labeling or tagging data with specific information, allowing AI models to recognize patterns, make predictions, and enhance decision-making processes. As AI development services and generative AI development services expand, the demand for precise and high-quality annotated data has never been higher.
Understanding Data Annotation
Data annotation is the process of labeling or tagging various forms of data—such as images, text, audio, and video—so that machines can understand and interpret this data. The annotated data serves as the ground truth for AI models during the training phase, helping them learn to identify objects, understand language, or recognize speech patterns, among other tasks.
Types of Data Annotation
Image Annotation:
-
Bounding Boxes: Drawing rectangles around objects in an image.
-
Semantic Segmentation: Labeling every pixel in an image with a corresponding category.
-
Keypoint Annotation: Marking specific points of interest, such as facial landmarks or body joints.
Text Annotation:
-
Named Entity Recognition (NER): Identifying entities like names, dates, or locations in text.
-
Sentiment Analysis: Labeling text based on the sentiment expressed, such as positive, negative, or neutral.
-
Part-of-Speech Tagging: Assigning grammatical categories to words in a sentence.
Audio Annotation:
-
Speech Recognition: Transcribing spoken words into text.
-
Speaker Identification: Identifying individual speakers in an audio file.
-
Sound Labeling: Tagging sounds or noises within an audio clip, such as applause or background music.
Video Annotation:
-
Object Tracking: Continuously labeling objects as they move through frames.
-
Action Recognition: Identifying specific actions or events within a video, such as running or jumping.
-
Scene Classification: Labeling different scenes or environments in a video.
Why is Data Annotation Important?
Enhances Machine Learning Accuracy
The quality and accuracy of annotated data directly impact the performance of AI models. Well-annotated data ensures that models can make precise predictions, reducing errors and improving reliability.
Enables Supervised Learning
Many AI and ML algorithms rely on supervised learning, which requires large amounts of labeled data to train models effectively. Data annotation provides this labeled data, enabling models to learn and generalize from examples.
Facilitates Real-World Applications
From autonomous vehicles to medical diagnostics, data annotation is critical in enabling AI applications to function in real-world scenarios. For example, annotated images help self-driving cars recognize road signs, pedestrians, and other vehicles.
Supports Natural Language Processing (NLP)
In NLP, data annotation allows models to understand and process human language. The annotated text helps in tasks like machine translation, chatbots, and sentiment analysis, making AI more interactive and user-friendly.
Improves Data Quality
Annotating data also helps in identifying and correcting inconsistencies, errors, or biases in datasets. This ensures that the data used for training is clean, accurate, and representative, leading to better model performance.
Challenges in Data Annotation
Time-Consuming and Labor-Intensive
Annotating large datasets can be a time-consuming process, requiring significant human effort. The complexity of the task increases with the amount of data and the level of detail required.
Subjectivity and Inconsistency
Different annotators might interpret the same data differently, leading to inconsistencies in the annotations. Ensuring a standard approach and maintaining consistency is crucial.
Cost
High-quality data annotation often involves specialized knowledge and expertise, which can be costly. Outsourcing to annotation services or using in-house teams can add to the overall project budget.
Scalability
As the need for annotated data grows, scaling the annotation process becomes a challenge. Balancing quality with quantity is essential to meet the demands of large-scale AI projects.
Why You Need Data Annotation
Critical for AI Development
Without annotated data, AI models cannot learn or make accurate predictions. Data annotation is the backbone of AI development, enabling machines to understand and interact with the world around them. AI consulting companies rely on high-quality data annotation to develop reliable models for various industries.
Customizing AI Solutions
Different industries require customized AI solutions that cater to specific needs. Data annotation allows for the creation of domain-specific models tailored to particular applications like healthcare, finance, or retail. AI development services leverage annotated data to create solutions that meet unique business requirements.
Enhancing User Experience
Annotated data improves the accuracy and relevance of AI-powered applications, leading to a better user experience. Whether it's personalized recommendations, virtual assistants, or smart devices, data annotation plays a crucial role in making AI more intuitive and responsive.
Staying Competitive
In a rapidly evolving technological landscape, businesses that leverage AI and ML effectively gain a competitive edge. Data annotation is essential for building robust AI models that drive innovation and keep businesses ahead of the curve. Generative AI development services, in particular, benefit from well-annotated data to produce innovative and creative solutions.
Conclusion
Data annotation is a fundamental process that underpins the success of AI and machine learning models. It transforms raw data into valuable insights, enabling machines to learn, predict, and make decisions with greater accuracy.
Despite its challenges, the benefits of data annotation make it indispensable for anyone looking to harness the power of AI. Whether you are developing cutting-edge technologies or improving existing systems, investing in high-quality data annotation is crucial to achieving your AI goals.