Overcoming Challenges in Generative AI Implementation
Apart from offering benefits like improving decision-making, enhancing customer satisfaction & diminishing risks, Gen AI deals with several implementation challenges that businesses often struggle with. This blog outlines the challenges associated with the implementation of Gen AI in businesses and possible strategies to mitigate those challenges.
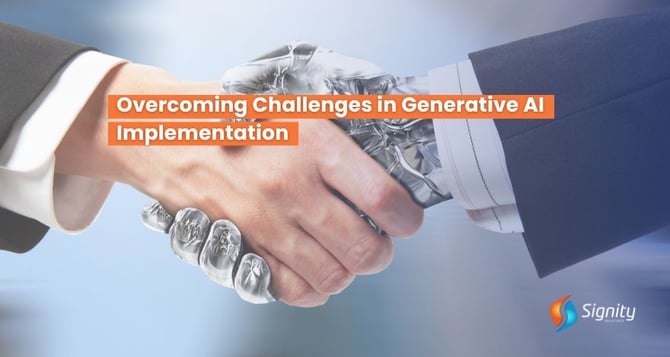
Generative AI holds huge potential for businesses across varied industries. From improving customer satisfaction to enhancing business efficiency with Gen AI and creativity and incorporating innovation, the advantages of adopting Gen AI are vast.
The world is full of examples of what generative AI can do, including ChatGPT, Google Bard, Bing Chat Midjourney, GitHub Co-Pilot, and Dall-E 2. Generative AI's adaptability in fusing various media forms—for example, turning text into images or audio into text—has opened up a wide range of imaginative and profitable possibilities.
Key Takeaways
- The challenges of generative AI can be overcome through a well-planned implementation approach, collaboration, and regulatory guidance.
- The future of generative AI is uncertain, as it has the potential to transform our personal and professional lives.
According to a recent survey of over 500 senior IT leaders, most of them (67%) plan to prioritize generative AI within the next 18 months for their company, with one-third (33%) saying it will be their top priority.
However, with so many possibilities, many companies are also hesitant to adopt or use this technology, even though it's becoming increasingly integrated into research and operational processes across diverse industries.
The reason?
Well, it is the challenge of implementing generative AI. Read on to learn about these challenges and get acquainted with strategies for overcoming them.
Understanding the Challenges
A July 2023 report by Goldman Sachs estimates that after ten years of widespread adoption, GenAI could increase productivity by 1.5 percentage points and boost the world GDP by 7%.
However, most executives will first need to overcome a few significant obstacles before that can occur. However, to overcome those challenges, it is important to understand them thoroughly.
Let us check out some of the challenges:
-
Data Security and Privacy
One of the crucial challenges that companies may encounter while implementing generative AI is data security and privacy.
Generative AI models rely heavily on huge data sets to create accurate and meaningful outcomes. However, handling such huge and sensitive data can pose privacy and security concerns.
-
Ethical and Bias Considerations
Gen AI models generally learn from the input data, and if the training data fed into the system is biased, the output delivered by the Gen AI models will also be biased. This bias in data may lead to unfair outcomes that may hinder the brand's reputation.
In a BCG report, over 80% of participants identified governance and a lack of a strategic roadmap as their biggest obstacles, and more than 70% of respondents are hesitant to use GenAI.
In businesses where transparency is crucial, biased results may become a major barrier to AI adoption. Therefore, it is crucial to address these ethical concerns to expect a sense of fairness in the outcomes.
Empower Your Innovation Journey with AI
Contact our team to explore tailored AI solutions that can boost your business growth.
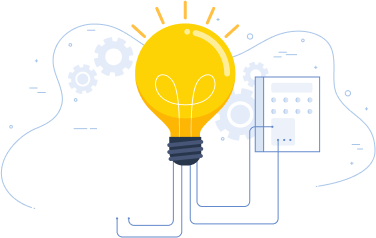
-
Computational Costs and Resources
Training generation AI models requires substantial computational resources, including high-performance GPUs and significant memory. However, midsized or small businesses may encounter challenges in training and deploying these AI models due to restricted access to high-performance computing resources.
For example, a startup that wants to deploy AI models to offer personalized product recommendations to its audience may not have the right infrastructure and computational power to train the AI models, limiting its ability to offer customized recommendations.
-
Integration With Existing Systems
Integrating the Gen AI technology with the existing business processes and workflow can be challenging and complicated.
It requires strong technical know-how and frequent modifications to enable the AI models to interact with the existing models. This may lead to resistance among the force.
-
Handling Technical Intricacies
Gen AI models include numerous parameters that make them complicated for businesses. These models are generally huge to train for most organizations.
A pharmaceutical company's director of commercial and IT claimed, "We lack access to the right set of skills—not only technical, but also skills associated with implementing the use cases, like legal, data privacy, and compliance."
The essential calculation of the resources required to implement this can make the technology ecologically unfriendly and expensive. Creating models to handle these technical intricacies can lead to other problems, like deep-pocketed entities.
Therefore, most businesses might likely adopt generative AI through cloud APIs with restricted tuning.
-
Transparency and Explainability
Generative AI models based on deep learning are often known as "black boxes" because their decision-making processes are complicated to understand.
Therefore, this lack of transparency acts as a huge barrier to acceptance and trust, both among customers and within the organization.
-
Data Quality and Quantity
Generative AI models need huge amounts of data to function appropriately. Several organizations struggle to obtain high-quality data that represents a particular product domain.
Without this foundational dataset, the outcomes of the AI models may lack accuracy and relevancy.
-
Fine-tuning and Customizations
Off the shelf, the Gen AI models might not suit the specific business requirements. Therefore, they require customization and fine-tuning by professionals with expertise in data science and machine learning.
-
Intellectual Property (IP) Issues
Data that is private or protected by copyright can also be used to train generative AI models. In that case, the ownership and use of the generated content might give rise to moral and legal issues.
Recommended Read: What is Generative AI? A Comprehensive Guide
Strategies for Overcoming Challenges
It's important to note that the chances of successfully solving generative AI implementation challenges are high. The image below illustrates that the likelihood of resolving AI challenges increases with each step.
Therefore, adopting and incorporating some of the strategies can mitigate the risk of challenge occurrence. Let us check out some of the strategies to overcome the challenges in generative AI implementation.
-
Compliance with Data Protection Laws and Ethical Guidelines
To handle data security and privacy concerns, businesses are required to ensure strict adherence to ethical guidelines and compliance with data protection laws to protect sensitive data from potential breaches.
Also, adopting robust data governance practices and policies, including data anonymization techniques and strong encryption methods, is crucial to maintaining compliance with regulations and standards in generative AI projects.
For instance, if any healthcare institution is required to incorporate generative AI models based on the medical records shared, they must anonymize their data to maintain data privacy while ensuring compliance with the standards and regulations.
-
Employ Unbiased Data Sets
When training AI models, businesses must use unbiased datasets and regularly test and monitor the generated outcomes to identify any biases or discrimination in the data.
Incorporating bias and ethical considerations into the AI implementation strategy is imperative.
For instance, if any insurance company wants to use general AI models that can automatically assess damages and calculate claims, they must train the implemented AI models with different datasets to generate unbiased output and provide a coherent explanation of the decision-making process.
-
Using Managed AI Services
Businesses or startups should consider using managed AI services to eliminate the need to manage model training and infrastructure. This enables businesses to leverage Gen AI's capabilities without worrying about computational requirements.
Consider cost optimization strategies, such as using spot instances for noncritical workloads or setting up scans based on workload requirements, to reduce costs.
-
Deploy Necessary Training
Businesses are required to create a change management strategy that includes necessary training to overcome employees' concerns. When providing the training, companies must emphasize the value Gen AI model incorporation can bring to individual roles and businesses.
-
Improvement in AI Model's Explainability
Several techniques, like local interpretable model agnostic explanations (LIME) and model interpretability, can be implemented to enhance the transparency and explainability of the gen AI model. Businesses can also partner with a model provider who prioritizes transparency to ensure trust and seamless communication.
-
Proof of Concept Creation
To avoid challenges related to artificial intelligence technology, it is recommended that you start your AI project with a discovery phase and create a proof of concept.
This will help you map out the solution requirements and align them with your business needs. Additionally, it will allow you to identify and eliminate any potential technology barriers and design the system architecture with the expected number of users in mind.
It is also crucial to select an AI technology partner with experience overcoming data-related challenges in artificial intelligence.
-
Adversarial Testing
Before becoming accessible to the general public, generative AI should undergo extensive testing in controlled settings. This enhances the user experience and streamlines the process of locating and fixing possible problems.
Real-World Examples of Gen AI Implementation
Several companies are now using generative AI to improve business operations. For example,
-
Delta Airlines
Delta Airlines is recognized for its dedication to enhancing customer experience and service. The airline has successfully integrated gen AI into customer experience. They have incorporated an AI chatbot called "Ask Delta," which assists customers by helping them find flights, check-in, and track their baggage.
The AI chatbot provides comprehensive responses to customers and offers the information they require. Since implementing this technology in its customer experience strategy, the airline has witnessed a 20% drop in call center volume.
Gen AI is also being employed by several other industries to enhance the customer experience.
-
Pharmaceuticals
Pharmaceutical companies like Amgen and Insilico Medicine, as well as academic researchers, are utilizing generative AI to design proteins for medicines. Predicting the folding of proteins has been a significant challenge for geneticists and pharmaceutical developers for decades. However, deep learning models such as generative adversarial networks (GANs) are enhancing researchers' abilities to comprehend and utilize protein synthesis.
-
Manufacturing
Manufacturing companies like Autodesk and Creo use generative AI technology to design physical objects. They often manufacture these objects through 3D printing or computer-controlled machining and additive manufacturing. Generative AI is capable of creating machine parts and subassemblies of larger objects while also optimizing designs for various aspects of the manufacturing process, such as materials efficiency, simplicity, and speed of production.
Generative AI technology improves the overall efficiency and effectiveness of the manufacturing process by minimizing waste, reducing the number of parts, and enhancing production speed.
-
Healthcare
Generative AI is used in healthcare for disease diagnosis, medication discovery, and medical image analysis, enabling faster drug discovery through pattern recognition.
Future Outlook and Recommendations
AI-powered tools have changed the way we interact with technology and are qualitatively different from all the tools of the past. We can talk to them, and they talk back. As they understand us better, they have become an integral part of our daily lives. They answer our questions, solve our problems, and even perform proofreading with impressive creativity that surpasses human efforts. They handle many of our tasks, and this integration is definitely going to increase in the future.
Various ways are being employed to employ AI currently. Here are some takeaways about how generative AI is changing the future of enterprises.
The Potential of Generative AI to Transform Software Interaction
Gen AI has the potential to revolutionize software usage. As more systems adopt it, user interaction and customer satisfaction should improve because these interfaces should be more personalized and intuitive, suited to each user's unique requirements and preferences.
Using Generative AI to Spark Business Transformation
AI will be a key factor in how businesses adjust their operations, business plans, and customer interactions to the current technological landscape. This could lead to more economical procedures that lower costs while raising productivity and creating new opportunities for study, growth, and innovation.
The use of Generative AI is expected to grow in the future and become more common in organizations. A recent poll conducted by Gartner, which surveyed over 1400 executive leaders, revealed that approximately 43% of respondents are currently testing generative AI tools and products.
Despite this trend, many executives remain skeptical about adopting AI due to common challenges. However, a well-defined generative AI implementation plan, smooth execution, and adoption of the above-mentioned strategies can mitigate the challenges and encourage wider and safer adoption of AI.
Bottom Line
It is crucial to understand that integrating generative AI into your organization is not a short-term IT project but rather a long-term strategic initiative requiring dedication, focus, and investment.
To ensure success, it is imperative to align the implementation of generative AI with your company's overall strategy instead of treating it as just another tech program. Attempting to run it on the sidelines without proper integration is likely to fail.
It's crucial to have a clear plan in place to achieve the desired outcomes while implementing an AI solution. However, this requires dedication and expertise.
Contact Signity's Generative AI development experts to turn your dream into a reality. Our AI experts possess the necessary expertise to help you implement your AI solution and bring your vision to life.