RAG vs. Traditional Search Engines: A Comparative Analysis
RAG provides precise, context-aware results by fusing AI with real-time data retrieval, outperforming traditional search. It enhances knowledge retention, provides context-aware replies, and dynamically adjusts to changing facts. This blog explores its benefits, use cases, and future impact.
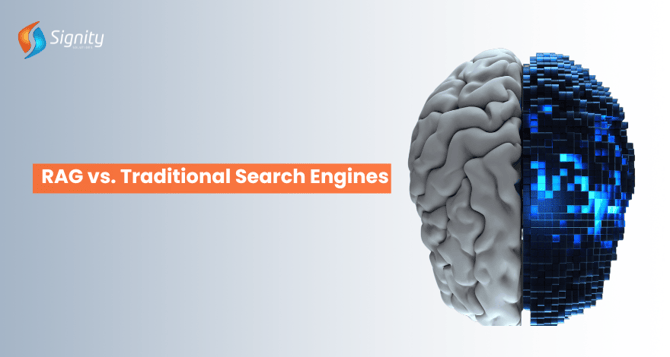
Retrieval-augmented generation (RAG) has become a powerful replacement for conventional search engines as a result of the considerable advancements in search technology. RAG combines generative AI with information retrieval to provide more contextually relevant and nuanced results than standard search, which depends on keyword matching and static databases.
With the right RAG AI services, you can build custom solutions to meet your business needs. By dynamically sourcing relevant information from both structured and unstructured sources, RAG supports the data retrieval process and guarantees contextual relevance and real-time accuracy.
Retrieval-augmented generation increases content creation, boosts knowledge retention, and allows AI-driven systems to produce more accurate answers to user inquiries.
This blog explores the differences between RAG and traditional search, their benefits, and future trends.


- RAG enhances search accuracy with large language models, context recall, and dynamic data retrieval, offering context-aware answers beyond keyword matching.
- Automated tuning, evaluation metrics, and query transformations help refine search performance and ensure correctness over time.
- RAG combines structured and unstructured data, improving search relevance with semantic similarity and dynamic knowledge updates.
- RAG's context-driven pipeline delivers up-to-date results, making it ideal for industries requiring real-time information.
Comparison of RAG and Traditional Search
For a long time, traditional search engines have retrieved relevant results by using indexed databases and keyword matching. However, search has changed into a more dynamic and context-aware procedure with the advent of the Retrieval-Augmented Generation.
How Traditional Search Engines Work
Traditional search engines use metadata embedding, static databases, and keyword matching to find information. They classify and rate outcomes using structured data. Link architectures and keyword density are the main determinants of the ranking algorithms.
Although these techniques guarantee the retrieval of a wide range of information, they frequently overlook user intent, producing irrelevant results.
How RAG Works
By combining real-time retrieval augmented generation with large language models (LLMs), RAG improves search and guarantees dynamic results. Retrieval-based and generative AI techniques are combined, and context recall and query modifications are used to increase accuracy.
In contrast to conventional search, RAG generates results that go beyond keyword-based queries by utilizing generative AI and dynamic knowledge upgrades.
The following table highlights the key differences between RAG and traditional search engines by analyzing the retrieval mechanisms, optimization processes, and entities present in both systems:
Feature |
Traditional Search Engines |
RAG Search |
Retrieval mechanism |
Keyword matching, static index |
Dynamic knowledge updates, context recall |
Understanding queries |
Limited to keywords |
Semantic similarity, query transformations |
Data sources |
Static databases |
Real-time information retrieval |
Response generation |
Pre-indexed results |
Generative AI responses |
Optimization |
Manual SEO and ranking adjustments |
Automated tuning, optimization tools |
Use of metadata |
Explicit metadata only |
Ontology-based, structured, and unstructured |
Adaptability |
Fixed algorithm-based ranking |
Continuous learning and tuning |
Benefits of RAG Over Traditional Search
By combining real-time retrieval augmented generation with large language models (LLMs), RAG improves search and guarantees dynamic results. Retrieval-based and generative AI techniques are combined, and context recall and query modifications are used to increase accuracy.
RAG is a more flexible search page solution than standard search since it incorporates generative AI and dynamic knowledge updates, which generate results beyond keyword-based searches.
Some key advantages of RAG Include:
1. Contextually Relevant Answers
Conventional search engines frequently provide irrelevant results since they only match keywords. However, RAG provides responses that are in line with the true meaning of the question since it comprehends the user's purpose and context. Accuracy and user satisfaction are greatly improved.
2. Real-Time Information Retrieval
RAG dynamically retrieves the most recent and pertinent material, in contrast to conventional search engines that depend on static databases. It is very useful in fields like banking, healthcare, and legal consulting, which need the most recent information.
3. Complex Data Integration
RAG can gather data from a variety of forms since it handles both structured and unstructured data sources well. RAG guarantees smooth integration and accessibility regardless of the data's storage format—documents, databases, or APIs.
4. Explicit Metadata Utilization
Search accuracy may be limited by traditional search engines' reliance on specified metadata. By using ontologies and schema-based classification, RAG improves search and guarantees accurate and contextually relevant results.
5. Creative Text Formats
RAG's generative AI capabilities enable it to provide responses in a variety of formats, including reports, summaries, and conversational responses. Businesses that need thorough explanations rather than just keyword-based search results may benefit from this flexibility.
6. Enhanced Performance
RAG continually enhances search quality by using evaluation metrics and automatic tuning strategies. Over time, it provides more precise and effective answers by examining previous queries and improving retrieval models.
7. Improved Context Recall
Context is difficult for traditional search engines to preserve over several inquiries. Better query refining and more meaningful interactions are made possible by RAG's capacity to remember pertinent information from prior searches, especially in research and customer service applications.
8. Better User Experience
RAG improves client satisfaction and engagement by providing responses that are more contextual, relevant, and organized. Its capacity to deliver clear and instructive responses lessens search frustration and boosts productivity.
Related read: Understanding RAG: Architecture, Techniques, Use Cases, & Development
Use Cases of RAG Pipeline
RAG is transforming various industries by enabling more intelligent and efficient search solutions:
- Healthcare: Through the use of natural language processing tools, RAG gives medical professionals access to the most recent research, clinical studies, and drug interactions. It facilitates quicker condition diagnosis and better patient care.
- Finance: Financial organizations use RAG for risk assessment, fraud detection, and transaction analysis. RAG facilitates speedy and precise decision-making by analyzing vast volumes of financial data.
- Legal Consulting: Law firms use RAG for compliance monitoring, case law research, and contract analysis. It guarantees accurate document retrieval and quicker legal insights through the use of ontology-driven vectors.
- E-Commerce: By studying behavioral data and comprehending user intent, RAG improves the shopping experience. Better search results, more individualized product recommendations, and an enhanced customer experience all result from this.
- Enterprise Knowledge Management: RAG is used by organizations to improve the usability and accessibility of internal knowledge repositories. It speeds up the process by which workers locate relevant data, policies, and documents, enhancing output and decision-making.
Implementation Insights and Best Practices
Optimizing retrieval pipelines is crucial to implementing RAG effectively. Ensuring high context recall improves relevance, while pre-trained models enhance accuracy. Experimenting with query transformations refines input structures, leading to better results. Monitoring evaluation metrics and fine-tuning search mechanisms ensures correctness. Balancing retrieval and generation is essential for optimizing structured data and generative AI.
1. Optimize Retrieval Pipelines
Optimizing the pipeline for efficiency is crucial to achieving high context recall for appropriate search. It entails improving query expansion, enhancing indexing tactics, and adding entity identification to increase accuracy. In order to guarantee smooth information retrieval while preserving real-time performance, AI teams also need to employ tuning strategies.
2. Enhance Accuracy with Pre-Trained Models
By utilizing large datasets, pre-trained models assist in producing accurate and transparent results. Prioritize entity recognition, semantic comprehension, and fine-tuning according to assessment measures to increase their efficacy. AI-driven search engines produce precise, context-aware results when retrieval and generation techniques are properly combined.
3. Experiment with Query Transformations
Retrieval accuracy is considerably increased by improving input structures. To enhance context comprehension, AI teams should experiment with query rewriting strategies that make use of entity linkage and language modeling. Decreasing false positives and increasing search relevance help improve pipeline efficiency.
4. Monitor Evaluation Metrics
To ensure accuracy and dependability, AI systems must be continuously evaluated using evaluation metrics. Frequent performance evaluations assist in pinpointing areas that require enhancement, and methodical tuning strategies guarantee that AI models maintain their accuracy. Retrieval pipelines are gradually optimized, and flexibility is increased through A/B testing and real-world feedback loops.
5. Balance Retrieval and Generation
Striking the right combination between structured search methods and generative AI is key to achieving optimal results. Effective AI-driven pipelines must integrate structured databases with generative models to ensure relevant, high-quality outputs. AI teams should fine-tune ranking algorithms and response generation techniques while maintaining a focus on precision and performance.
Future of Search Technologies for Business Teams
AI-driven models, such as OpenAI's SearchGPT, are revolutionizing search by facilitating expressive inputs and ongoing learning. The AI revolution is improving expert knowledge and foundation models, and schema-driven search technologies are being integrated into enterprise content management systems. Companies that use continuous inquiries will see improved performance and more individualized outcomes.
Discover Smarter Search for Your Business With RAG
Improve accuracy, knowledge retention, and user experience with our solutions.
This development enhances decision-making by enabling business teams to obtain pertinent information more quickly. Additionally, it saves time spent looking for important insights, which aids firms in streamlining procedures.
Find Smarter Solutions with Signity’s RAG-Powered AI
At Signity, we use Retrieval-Augmented Generation (RAG) to help companies create more intelligent, contextually aware, and accurate search experiences. Our RAG solutions combine real-time data retrieval with big language models, giving your company accurate insights, dynamic knowledge updates, and improved user engagement to keep it ahead of the competition.
Whether you want to enhance customer service, enterprise knowledge management, or data-driven decision-making, our AI-driven RAG services offer the efficiency, flexibility, and optimization you want. Join forces with Signity to open the door to intelligent search and information retrieval in the future.
Frequently Asked Questions
Have a question in mind? We are here to answer. If you don’t see your question here, drop us a line at our contact page.
What is Retrieval-Augmented Generation (RAG), and how does it differ from traditional search engines?
Why is RAG considered more effective than traditional search engines?
What are the key benefits of using RAG for businesses?
Can RAG be integrated into existing enterprise search systems?
What does the future hold for search technologies with RAG?