Trends in Active Retrieval Augmented Generation: 2025 and Beyond
RAG is advancing AI with real-time retrieval, hybrid search, and multimodal capabilities. Trends like personalized RAG, on-device AI, and scalable solutions will impact industries. This blog explores RAG’s future and its business potential in 2025 and beyond.
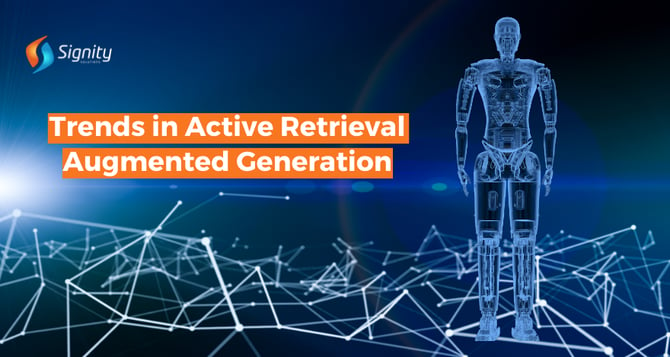
Retrieval-Augmented Generation (RAG) is gaining traction due to its ability to enhance the accuracy and contextual understanding of AI-generated content. Businesses leveraging RAG benefit from improved efficiency optimization, enhanced data curation, and better compliance with regulatory requirements.
By integrating data management systems and robust data pipelines, organizations can refine their generative AI applications and retrieve relevant information from multiple data sources.
RAG services optimize AI responses to user queries by retrieving relevant data based on the input query. From document search to AI-driven support, they enhance accuracy, relevance, and efficiency in content generation.
However, implementing RAG comes with challenges.
The computational expense of running large-scale RAG models can be significant, particularly when dealing with real-time latency constraints. Ensuring sensitive data security is another crucial concern, as RAG systems interact with vast repositories of technical documentation, graph databases, and unstructured data.
This blog will help you explore the future trends in RAG, its integration, and RAG applications in various industries.


- Real-time and Hybrid RAG: AI will retrieve the latest information dynamically using real-time feeds and hybrid search techniques, improving accuracy and relevance.
- Multimodal and Personalized AI: RAG will evolve beyond text, integrating images, videos, and audio while delivering highly personalized content through advanced fine-tuning.
- On-device and efficient Retrieval: AI models will process data locally for better privacy and reduced latency, while sparse retrieval techniques enhance speed and efficiency.
- RAG’s industry Impact: From legal and healthcare to finance and e-commerce, RAG will power precise decision-making, automation, and improved AI-driven insights.
Future Trends and Innovations in RAG
Emerging advances in Retrieval-Augmented Generation (RAG) are expected to improve AI's capacity to retrieve and produce precise, context-aware material. The future of AI-driven knowledge systems will be shaped by innovations such as hybrid search, multimodal RAG, and real-time retrieval.
1. Real-Time RAG
AI systems will be able to dynamically retrieve the most recent information by integrating real data feeds into RAG models. Real-time RAG will guarantee that generative AI solutions deliver precise and contextually appropriate material by establishing connections with external knowledge bases, websites, and structured data sources.
Companies will use this capability to improve customer involvement and make choices, particularly in sectors that need constant data updates.
2. Hybrid Models
The retrieval process will be optimized by combining keyword search with sophisticated retrieval techniques like knowledge graphs and semantic search. By obtaining pertinent documents from many data sources, optimizing search results, and increasing response accuracy, hybrid models will improve AI applications.
Information retrieval systems that analyze large datasets efficiently and relevantly will find this method especially helpful.
3. Multimodal Content
For a more comprehensive AI-driven experience, RAG will develop beyond text-based retrieval to include photos, videos, and audio. AI systems will be able to evaluate and retrieve data from a variety of external sources by utilizing vector databases and hybrid retrieval techniques.
The overall user search experience will be improved by this innovation, which will increase AI's capacity to adapt to different information forms.
4. Personalized RAG Implementation
AI models will be able to retrieve and produce highly personalized content thanks to developments in fine-tuning approaches like few-shot prompting and low-rank adaptation (LoRA). Customized RAG will improve customer interactions, obtain pertinent data based on context, and refine user questions.
Applications such as AI-powered customer service, tailored suggestions, and adaptive learning systems will benefit greatly from these capabilities.
5. On-Device AI
More RAG implementations will operate locally on user devices in response to the increasing desire for privacy and decentralized processing. By doing this, users will be able to process and retrieve data from their own data repositories, reducing their dependency on cloud-based retrieval techniques.
By enabling real-time information retrieval without external data access, on-device AI will also improve data security and reduce latency.
6. Sparsity Techniques
Sparse retrieval models and effective data architecture will enhance the retrieval system, lowering processing costs and guaranteeing quicker search results. These methods will improve AI applications in large-scale sectors, including cybersecurity, healthcare, and finance, where quick information retrieval is essential.
7. Active Retrieval-Augmented Generation
AI models will use sophisticated retrieval techniques like semantic search, vector search, and graph embeddings to extract pertinent documents and outside information sources proactively. Artificial intelligence (AI) applications will provide increasingly accurate and contextually rich content by continuously improving their retrieval processes.
8. RAG as a Service
Businesses will be able to deploy scalable, affordable RAG architectures thanks to cloud-based RAG solutions. Organizations may maximize their AI capabilities, expedite data access, and incorporate AI-powered retrieval systems into their workflows without having to make large infrastructure investments by implementing RAG as a Service.
9. Advancements in RAG Architecture
Enhancing information retrieval efficiency, integrating numerous data sources, and maximizing AI model performance will be the main goals of ongoing research and development in retrieval-augmented generation work. Businesses can produce AI-generated content that is more accurate and efficient by optimizing retrieval workflows and enhancing external knowledge integration.
10. Enhanced RAG Pipeline
Future advancements will improve the way AI models pull pertinent data from external data sources in the retrieval mechanism. Better retrieval augmentation techniques can further streamline the retrieval process and guarantee that AI-generated answers are based on the most up-to-date and accurate data.
RAG Applications in Various Industries
Retrieval-augmented generation (RAG) is improving AI-driven knowledge retrieval and decision-making, revolutionizing a number of industries. Companies can deliver precise, up-to-date information in a variety of domains by leveraging RAG-powered insights.
1. Legal Tech
Faster legal research and precedent identification are made possible by AI-driven case law analysis, which helps attorneys present more compelling arguments. Inconsistencies and compliance hazards are found via automated contract review, which guarantees that documents adhere to legal requirements. AI-powered regulatory updates also assist law firms in keeping up with changing legal regulations.
2. Healthcare
AI-assisted clinical decision support helps physicians make well-informed decisions by retrieving the most recent medical guidelines. By examining past medical records and present medical problems, personalized treatment recommendations enhance patient care. Additionally, AI-powered medical billing and coding improves accuracy while lessening the administrative burden on healthcare practitioners.
3. Finance
Real-time financial risk prevention is achieved using AI-driven fraud detection, which finds transaction irregularities. Automated investing insights examine consumer preferences and market developments to provide tailored financial solutions. Additionally, regulatory compliance monitoring driven by AI guarantees that companies easily comply with evolving financial requirements.
Related read: Check out more about RAG in financial services
4. Customer Service
Chatbots and virtual assistants driven by AI improve client engagement by responding with precision and personalization. Self-service assistance is improved by knowledge base automation, which provides rapid access to pertinent information. Businesses can have more successful and sympathetic relationships with customers by using sentiment analysis to further customer engagement and better understand their feelings.
5. E-commerce & Retail
AI-powered product suggestions use consumer behavior analysis to tailor shopping experiences. In order to maintain competitive positioning, dynamic pricing optimization modifies prices in response to market movements. AI-powered inventory forecasting also assists companies in maintaining ideal stock levels, avoiding shortages or surpluses.
6. Education & E-learning
Adaptive learning driven by AI improves learning results by customizing instructional materials to each student's needs. By including the most recent information, automated content curation ensures that course materials remain current. AI-powered real-time tutoring offers immediate assistance, enabling students to get help whenever they need it.
7. Manufacturing and Supply Chain
Predictive maintenance driven by AI stops equipment failures by seeing possible problems before they happen. By tracking shipments and forecasting demand, real-time supply chain monitoring improves logistics. Defect detection is guaranteed by AI-driven quality control analysis, increasing the overall dependability of the product.
Integration of Retrieval-Augmented Generation (RAG)
RAG is becoming a fundamental component in AI systems, improving how large language models (LLMs) interact with external knowledge sources. Innovations like real-time retrieval augmented generation and RAG-streaming will enable large language models with seamless data integration.
1. Adaptive Retrieval
Traditional search techniques' dependence on static retrieval procedures makes them ineffective for complicated queries. By dynamically adapting to query complexity, adaptive retrieval techniques improve search procedures and guarantee that AI systems return the most pertinent and superior material.
AI-powered retrieval engines can increase the accuracy and efficiency of system searches by better understanding user intent through the use of semantic search, vector search, and hybrid search.
2. Hybrid Search
By merging structured and unstructured data sources, hybrid search techniques will maximize data extraction. To improve retrieval operations, these techniques use graph embeddings, vector databases, and keyword-based search.
Hybrid search strategies guarantee thorough and accurate information retrieval in sectors like banking and healthcare, where AI models require access to both structured records and unstructured textual material.
3. Knowledge Graphs and Graph Embeddings
The comprehension and retention of contextual information by AI applications will be improved via knowledge graphs and graph embeddings. These technologies will allow AI algorithms to obtain interconnected insights rather than discrete facts by mapping relationships between data pieces.
It will be especially helpful in enterprise knowledge management platforms, chatbots, and AI-driven decision-making systems where it's critical to preserve context over several interactions.
4. Multimodal RAG for Enhanced AI Interactions
Beyond text-based AI models, retrieval-augmented generation has a bright future. Multimodal RAG will include a variety of data formats, such as audio, video, and image, into AI-powered systems. By adding picture retrieval, speech-to-text conversion, and video analysis to their retrieval pipelines, AI assistants, chatbots, and content production models will be able to offer more comprehensive responses.
AI-powered systems will provide more interesting and educational user experiences as companies embrace multimodal retrieval.
5. Self-Querying RAG Models
AI systems are developing the ability to refine their search queries on their own. In order to obtain more pertinent information, self-querying RAG models will automatically evaluate and reword search queries. These models will iteratively improve query precision, reduce noise, and improve output quality by utilizing low-rank adaptation (LoRA), context-aware prompting, and few-shot prompting.
Applications such as AI-powered research assistants, customer service bots, and compliance monitoring systems would particularly benefit from this.
6. RAG as a Service
RAG in Edge Computing will be essential to enabling decentralized AI as worries over latency and data privacy increase. On-device artificial intelligence (AI) will improve real-time decision-making, lower fraud exposure, and lessen regulatory compliance concerns by processing data locally on devices rather than depending on cloud-based retrieval.
It will be especially important for sectors that handle sensitive data, like cybersecurity, healthcare, and finance, where data privacy is crucial.
RAG Process and Mechanisms
The RAG pipeline consists of several critical stages, ensuring that AI can retrieve and generate accurate information efficiently:
-
Document Processing: Data ingestion and structuring using dual-encoder architecture and knowledge bases.
-
Query Processing: Transforming user queries into machine-readable formats via semantic search and keyword search.
-
Retrieval: Using vector search and knowledge graphs to extract relevant data from external data sources and training data.
-
Context Assembly: Integrating retrieved data into the generation process using advanced retrieval methods.
-
Generation: Producing AI-driven responses with optimized RAG-token and RAG-sequence techniques, leveraging the latest AI technology.
Observability and AI readiness will be essential in ensuring the smooth implementation of these processes. Proper access control mechanisms will also be crucial for safeguarding sensitive data and source documents.
Enhance AI with Signity
At Signity, we help businesses stay ahead in the evolving landscape of Retrieval-Augmented Generation (RAG). Our expertise in real-time retrieval, multimodal RAG, and hybrid retrieval techniques ensures that your AI solutions are contextually aware and industry-specific.
Maximize Business Efficiency With Active RAG
Explore how AI-driven retrieval can enhance productivity, accuracy, and customer experience.
By seamlessly integrating RAG with external data sources, we enable you to leverage massive datasets for more accurate and relevant content generation.
With a focus on enhanced security, efficiency optimization, and cutting-edge AI advancements, Signity empowers organizations to gain a competitive edge with knowledge-driven AI solutions. Let us help you unlock the full potential of RAG for your business.
Frequently Asked Questions
Have a question in mind? We are here to answer. If you don’t see your question here, drop us a line at our contact page.
What is Retrieval-Augmented Generation (RAG), and how does it work?
RAG is an AI framework that enhances generative models by retrieving relevant external data before generating responses. It improves contextual accuracy by integrating structured and unstructured data sources, ensuring AI-generated content remains precise and up-to-date.
What are the key benefits of using RAG in AI applications?
What challenges do businesses face when implementing RAG?
What are the emerging trends in RAG technology for 2025 and beyond?
How can businesses effectively implement RAG in their AI workflows?